Amazon Comperehend
- Natural Language Processing and Text Analytics
- Input social media, emails, web pages, documents, transcripts, medical records (Comprehend Medical)
- Extract key phrases, entities, sentiment, language, syntax, topics, and document classifications
- Events detection
- PII Identification & Redaction
- Targeted sentiment (for specific entities)
- Compose
- Entities: Noun with Category, Confidence
- Key phrases: Noun
- Language
- Sentiment: Neutral, Positive, Negative, Mixed
- Syntax: Noun, Verb, Adposition, Adjustive, …
Amazon Translate
- Uses deep learning for translation
- Supports custom terminology
- In CSV or TMX format
- Appropriate for proper names, brand names, etc.
Amazon Transcribe
- Speech to text
- Input in FLAC, MP3, MP4, or WAV, in a specified language
- Streaming audio supported (HTTP/2 or WebSocket)
- Speaker Identificiation
- Specify number of speakers
- Channel Identification
- i.e., two callers could be transcribed separately
- Merging based on timing of “utterances”
- Automatic Language Identification
- Custom Vocabularies
- Vocabulary Lists (just a list of special words – names, acronyms)
- Vocabulary Tables (can include “SoundsLike”, “IPA”, and “DisplayAs”)
- Practices
- Call Analytics
- Medical
- Subtitling
Amazon Polly
- Neural Text-To-Speech
- Lexicons
- Customize pronunciation of specific words & phrases
- Example: “World Wide Web Consortium” instead of “W3C”
- SSML
- Speech Synthesis Markup Language
- Gives control over emphasis, pronunciation, breathing, whispering, speech rate, pitch, pauses.
- Speech Marks
- metadata can encode when sentence / word starts and ends in the audio stream
- Useful for lip-synching animation
Amazon Rekognition
- Computer vision
- Object and scene detection
- Image moderation
- Facial analysis
- Celebrity recognition
- Face comparison
- Text in image
- Video analysis
- Objects / people / celebrities marked on timeline
- People Pathing
- The Nitty Gritty
- Images come from S3, or provide image bytes as part of request
- Facial recognition depends on good lighting, angle, visibility of eyes, resolution
- Video must come from Kinesis Video Streams
- H.264 encoded
- 5-30 FPS
- Favor resolution over framerate
- Can use with Lambda to trigger image analysis upon upload
- Can use Custom Labels
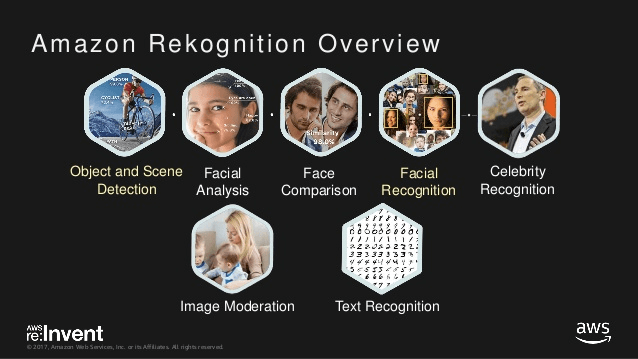
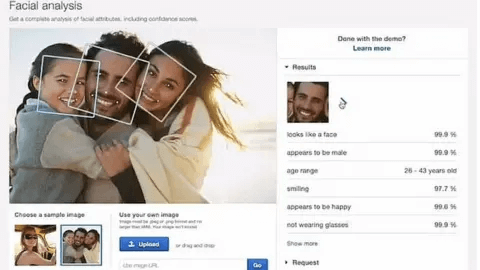
Amazon Forecast
- “AutoML” chooses best model for your time series data
- CNN-QR
- Convolutional Neural Network – Quantile Regression
- Best for large datasets with hundreds of time series
- Accepts related historical time series data & metadata
- DeepAR+
- Recurrent Neural Network
- Best for large datasets
- Accepts related forward-looking time series & metadata
- Prophet
- Additive model with non-linear trends and seasonality
- NPTS
- Non-Parametric Time Series
- Good for sparse data. Has variants for seasonal / climatological forecasts
- ARIMA
- Autoregressive Integrated Moving Average
- Commonly used for simple datasets (<100 time series)
- ETS
- Exponential Smoothing
- Commonly used for simple datasets (<100 time series)
- CNN-QR
- Works with any time series
- Price, promotions, economic performance, etc.
- Can combine with associated data to find relationships
- Inventory planning, financial planning, resource planning
- Based on “dataset groups,” “predictors,” and “forecasts.”
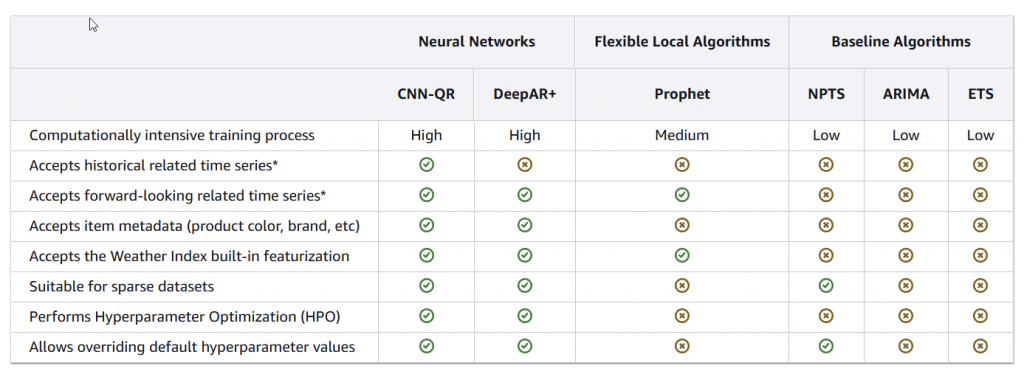
Amazon Lex
- Natural-language chatbot engine
- A Bot is built around Intents
- Utterances invoke intents (“I want to order a pizza”)
- Lambda functions are invoked to fulfill the intent
- Slots specify extra information needed by the intent
- Pizza size, toppings, crust type, when to deliver, etc.
- Can deploy to AWS Mobile SDK, Facebook Messenger, Slack, and Twilio
- Lex Automated Chatbot Designer
- provide existing conversation transcripts
- Lex applies NLP & deep learning, removing overlaps & ambiguity
- Intents, user requests, phrases, values for slots are extracted
- Ensures intents are well defined and separated
- Integrates with Amazon Connect transcripts
Amazon Personalise
- Fully-managed recommender engine
- API access
- Feed in data (purchases, ratings, impressions, cart adds, catalog, user demographics etc.) via S3 or API integration
- provide an explicit schema in Avro format
- Javascript or SDK
- GetRecommendations
- Recommended products, content, etc.
- Similar items
- GetPersonalizedRanking
- Rank a list of items provided
- Allows editorial control / curation
- Features
- Real-time or batch recommendations
- Recommendations for new users and new items (the cold start
problem) - Contextual recommendations
- Device type, time, etc.
- Similar items
- Unstructured text input
- Intelligent user segmentation
- For marketing campaigns
- Business rules and filters
- Promotions
- Inject promoted content into recommendations
- Can find most relevant promoted content
- Trending Now
- Personalized Rankings
- Terminology
- Datasets
- Users, Items, Interactions
- Recipes
- USER_PERSONALIZATION
- PERSONALIZED_RANKING
- RELATED_ITEMS
- USER_SEGMENTATION
- Solutions
- Trains the model
- Optimizes for relevance as well as your additional objectives
- Video length, price, etc. – must be numeric
- Hyperparameter Optimization (HPO)
- Campaigns
- Deploys your “solution version”
- Deploys capacity for generating real-time
recommendations
- Datasets
- Hyperparameter
- User-Personalization, Personalized-Ranking
- hidden_dimension (HPO)
- bptt (back-propagation through time – RNN)
- recency_mask (weights recent events)
- min/max_user_history_length_percentile (filter out robots)
- exploration_weight 0-1, controls relevance
- exploration_item_age_cut_off – how far back in time you go
- Similar-items
- item_id_hidden_dim (HPO)
- item_metadata_hidden_dim (HPO with min & max range specified)
- User-Personalization, Personalized-Ranking
- Maintaining Relevance
- Use PutEvents operation to feed in real-time user behavior
- Retrain the model
- They call this a new solution version
- Updates every 2 hours by default
- Should do a full retrain (trainingMode=FULL) weekly
Amazon TextTract
- OCR with forms, fields, tables support
AWS DeepRacer
- Reinforcement learning powered 1/18-scale race car
Amazon Lookout
- Equipment, metrics, vision
- Detects abnormalities from sensor data automatically to detect equipment issues
- Monitors metrics from S3, RDS, Redshift, 3rd party SaaS apps
- Vision uses computer vision to detect defects in silicon wafers, circuit boards, etc.
Amazon Monitron
- End to end system for monitoring industrial equipment & predictive maintenance
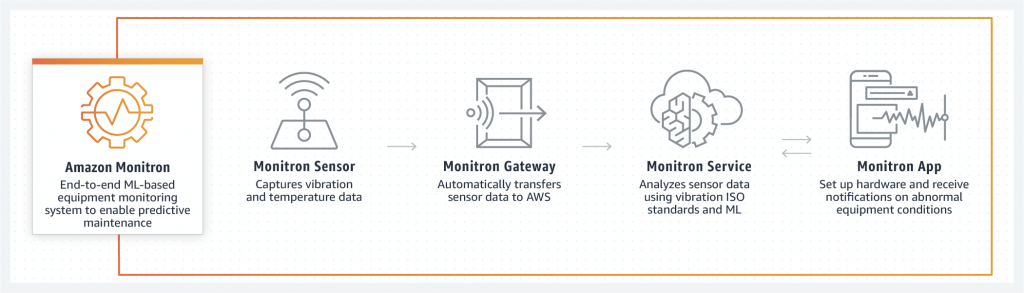
TorchServe
- Model serving framework for PyTorch
- Part of the PyTorch open source project from Facebook (Meta?)
AWS Neuron
- SDK for ML inference specifically on AWS Inferentia chips
- EC2 Inf1 instance type
- Integrated with SageMaker or whatever else you want (deep learning AMI’s, containers, Tensorflow, PyTorch, MXNet)
AWS Panorama
- Computer Vision (SDK) at the edge
- Brings computer vision to your existing IP cameras
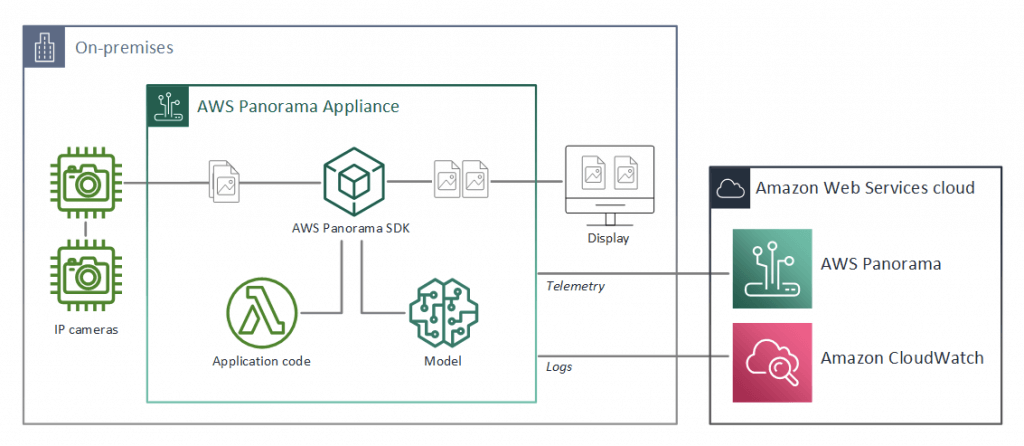
AWS Deep Composer
- AI-powered (music) keyboard
- Composes a melody into an entire song
- For educational purposes
Amazon Fraud Detection
- Upload your own historical fraud data
- Builds custom models from a template you choose
- Exposes an API for your online application
- Assess risk from:
- New accounts
- Guest checkout
- “Try before you buy” abuse
- Online payments
Amzon CodeGuru
- Part of CICD
- Automated code reviews!
- Finds lines of code that hurt performance
- Resource leaks, race conditions
- Fix security vulnerabilities
- Offers specific recommendations
- Powered by ML
- Supports Java and Python
Amazon Kendra
- Enterprise search with natural language
- Combines data from file systems, SharePoint, intranet, sharing services (JDBC, S3) into one searchable repository
- ML-powered (of course) – uses thumbs up / down feedback
- Relevance tuning – boost strength of document freshness, view counts, etc.
Use Cases | Amazon Kendra | Elasticsearch | OpenSearch |
Improve search experiences | Yes | Yes | Yes |
Enhance customer interactions and satisfaction | Yes | Yes | Yes |
Natural Language Processing (NLP) | Yes | Yes, but with the help of additional configurations or integrations. | Yes, but it requires external NLP tools. |
Full-text search | Yes | Yes | Yes, |
Enterprise search | Yes | Yes, but it requires extra configuration. | Yes, but it needs extra configuration. |
Distributed search | Yes | Yes | Yes |
Piped query language search | No | Yes | Yes |
Integrate search functionality into your SaaS applications | Yes | Yes | Yes |
Implement multi-tenancy and get rid of fraud and risk | Yes | Yes | Yes |
Semantic search | Yes | Yes | Yes |
Application search | Yes | Yes | Yes |
E-commerce search | No | Yes | Yes |
Log and event data monitoring | No | Yes | Yes |
Aggregate and analyze large datasets | No | Yes | Yes |
Security Information and Event Management (SIEM) | No | Yes | Yes |
Amazon Augmented AI (A2I)
- Human review of ML predictions
- Builds workflows for reviewing low-confidence predictions
- Access the Mechanical Turk workforce or vendors
- Integrated into Amazon Textract and Rekognition
- Integrates with SageMaker
- Difference with SageMaker Ground Truth
- Focus:
- A2I: Focuses on integrating human review into the decision-making process of models post-prediction.
- Ground Truth: Focuses on creating high-quality labeled datasets during the training phase.
- Stage of ML Workflow:
- A2I: Used during the model deployment and prediction phase.
- Ground Truth: Used during the data preparation and model training phase.
- Automation:
- A2I: Adds human review when machine confidence is low.
- Ground Truth: Combines automated labeling with human corrections to produce accurate training data.
- Focus:
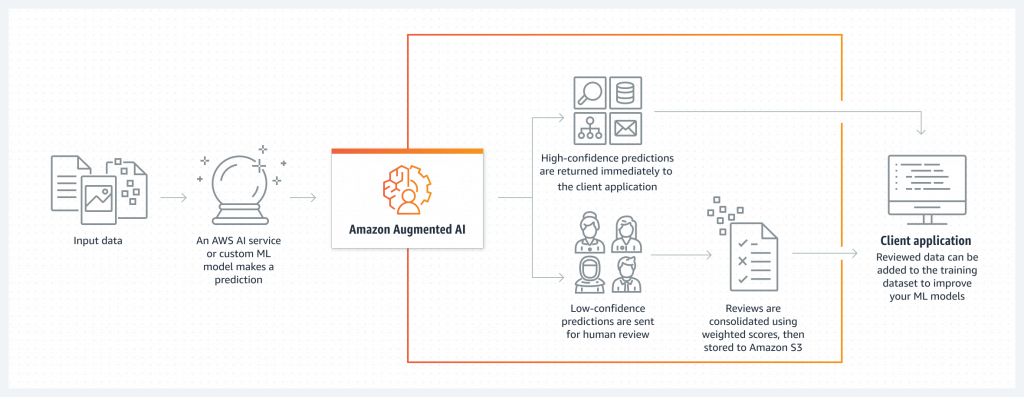
Amazon Connect
- an Amazon Web Services (AWS) public cloud customer contact center service
- Contact Lens
- For customer support call centers
- Ingests audio data from recorded calls
- Allows search on calls / chats
- Sentiment analysis
- Find “utterances” that correlate with successful calls
- Categorize calls automatically
- Measure talk speed and interruptions
- Theme detection: discovers emerging issues
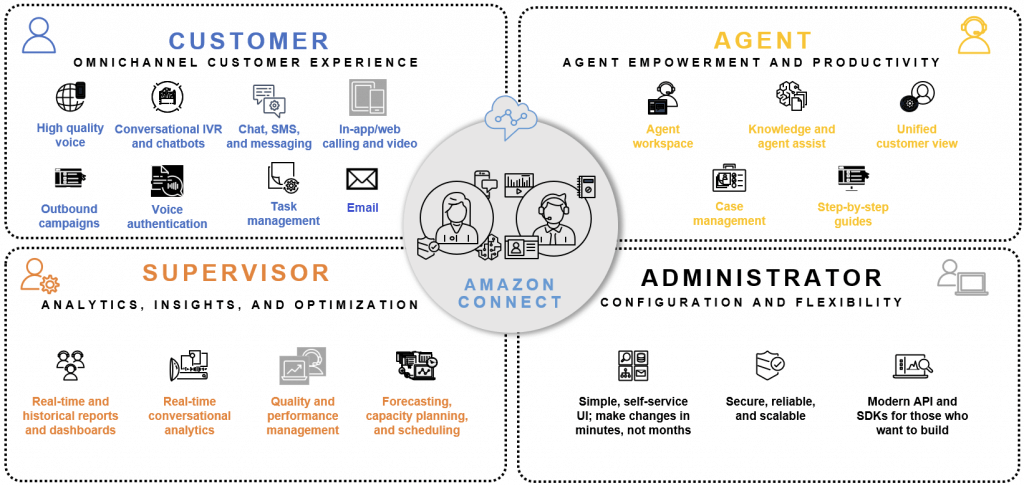