Machine Learning
- the process of training computers, using math and statistical processes, to find and recognize patterns in data
- After patterns are found, ML generates and updates training models to make increasingly accurate predictions and inferences about future outcomes based on historical and new data
- Process involved
- Data collection and preparation: Gathering relevant data and preprocessing it (cleaning, formatting, feature engineering) to make it suitable for model training.
- Model training: Feeding the prepared data into machine learning algorithms, which learn patterns and relationships within the data to build a model.
- Model evaluation: Assessing the trained model’s performance using evaluation metrics and techniques like cross-validation.
- Model deployment: Integrating the trained and evaluated model into applications or systems to make predictions or decisions on new data.
- Simple and complex ML models differ when balancing a model’s accuracy (number of correctly predicted data points) and a model’s explainability (how much of the ML system can be explained in “human terms”).
- The output of a simple ML model ( decision trees or logistic regression, for example) may be explainable and produce faster results, but the results may be inaccurate.
- The output of a complex ML model may be accurate, but the results may be difficult to communicate.
- Key words
- churn rate, sometimes known as attrition rate, is the rate at which customers stop doing business with a company over a given period of time. Churn may also apply to the number of subscribers who cancel or don’t renew a subscription. The higher your churn rate, the more customers stop buying from your business.
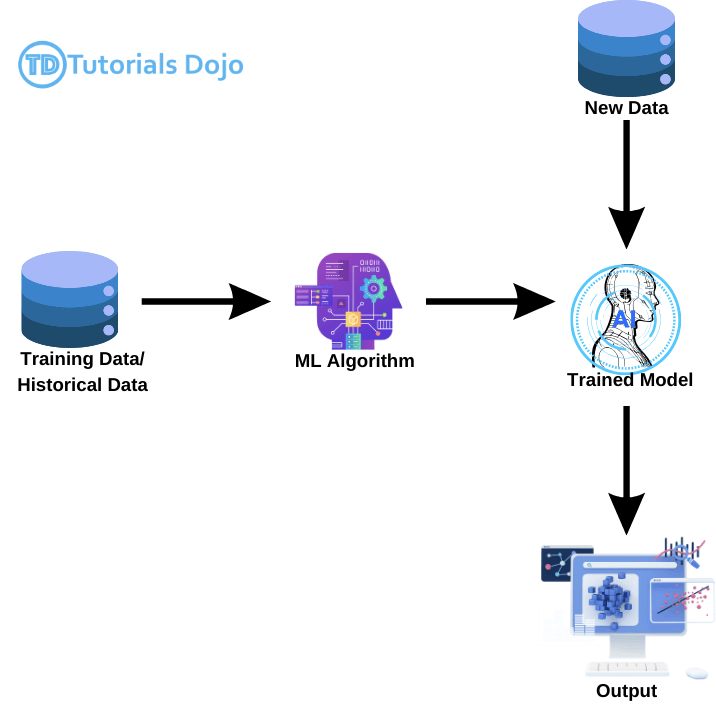
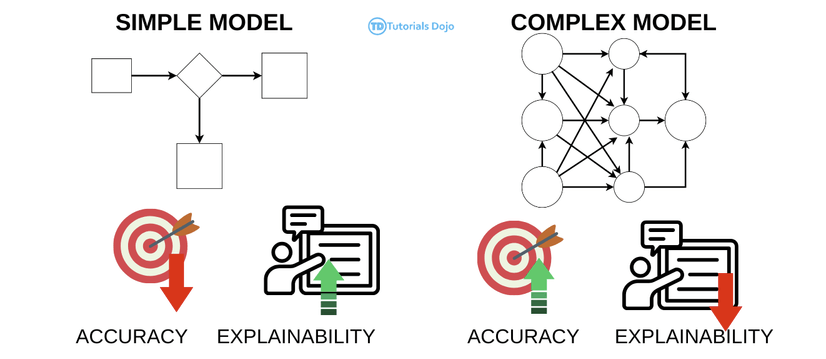
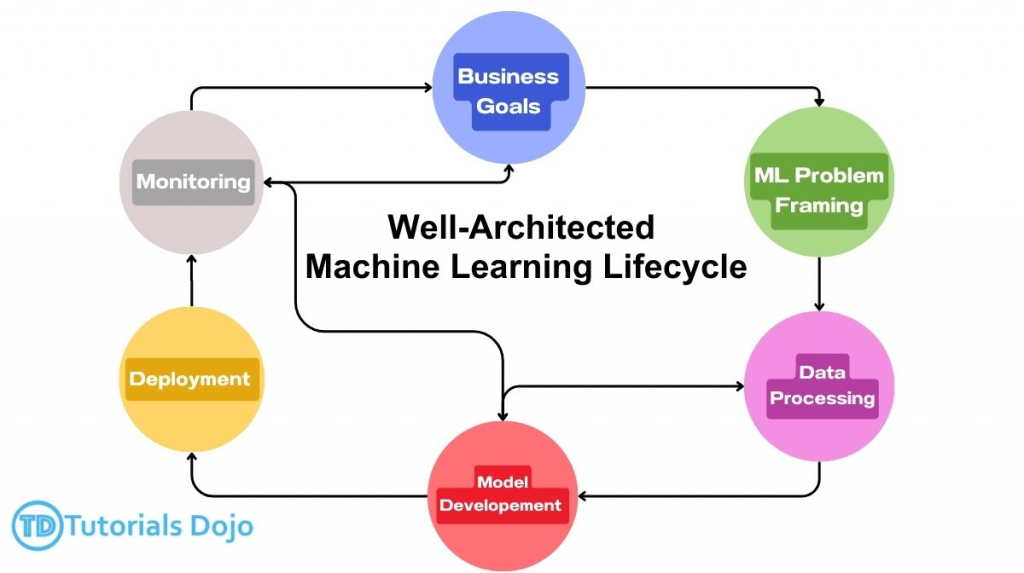
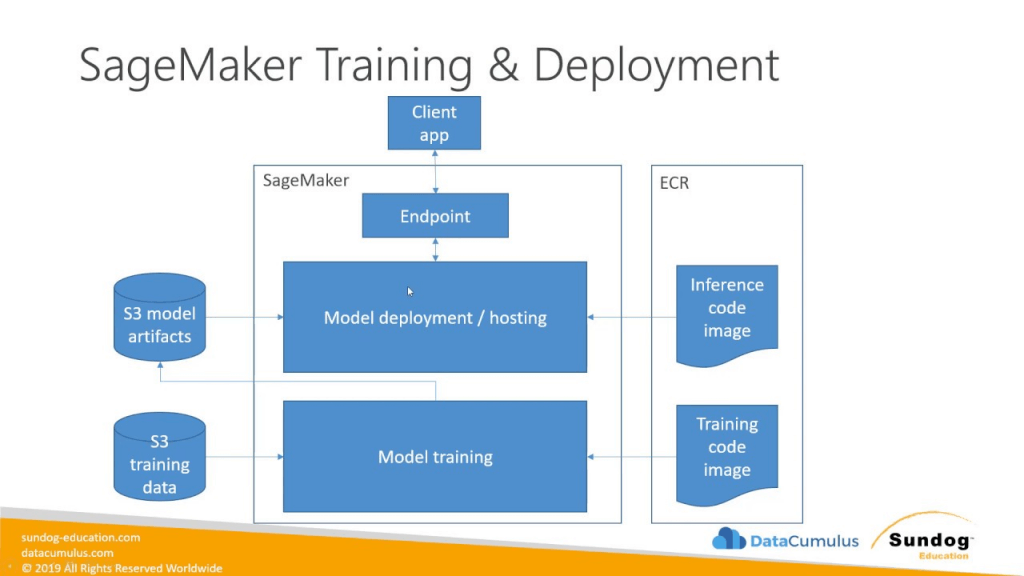
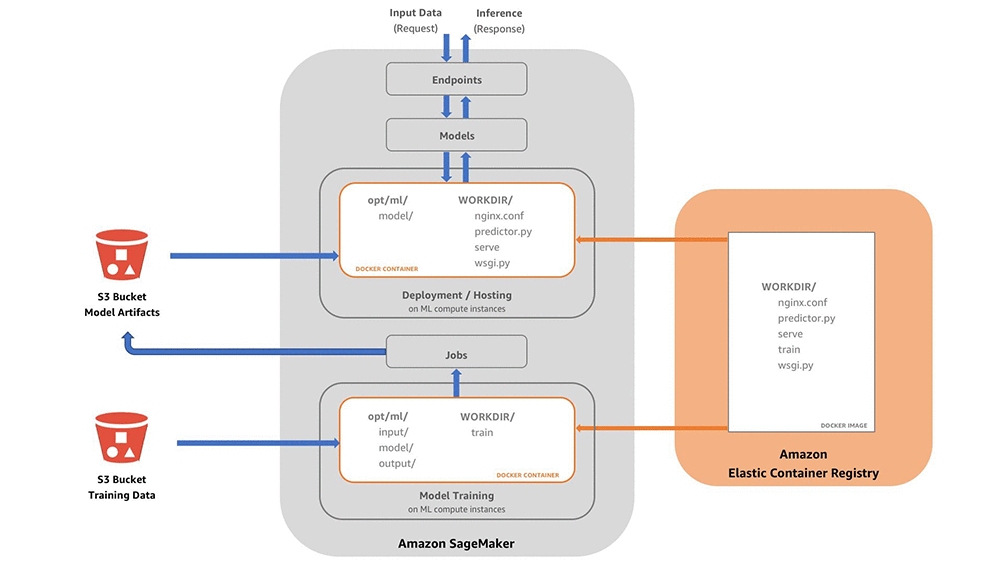
== COMPONENTS ==
SageMaker Notebooks
- direct the process
- (Jupyter) Notebook Instances on EC2 are spun up from the console
- S3 data access
- using Scikit_learn, Spark, Tensorflow
- Wide variety of built-in models
- Ability to spin up training instances
- Ability to deploy trained models for making predictions at scale
- notebook instances come with a pre-installed R kernel, which includes the reticulate library. This library provides an R to Python interface, enabling you to utilize the features of the SageMaker Python SDK directly within an R script. But it’s not designed for the production deployment of models.
- to fully running R models, please use custom R docker container with a SageMaker endpoint.
- LifeCycle Configuration
- A lifecycle configuration (LCC) provides shell scripts that run only when you create the notebook instance or whenever you start one. When you create a notebook instance, you can create a new LCC or attach an LCC that you already have. Lifecycle configuration scripts are useful for the following use cases:
- Installing packages or sample notebooks on a notebook instance
- Configuring networking and security for a notebook instance
- Using a shell script to customize a notebook instance
- You can also use a lifecycle configuration script to access AWS services from your notebook. For example, you can create a script that lets you use your notebook to control other AWS resources, such as an Amazon EMR instance.
- With the Lifecycle configuration feature in Amazon SageMaker, you can automate these customizations to be applied at different phases of the lifecycle of an instance. For example, you can write a script to install a list of libraries and, using the Lifecycle configuration feature, configure the scripts to automatically execute every time your notebook instance is started. Similarly, you can choose to automatically run the script only once when the notebook instance is created.
- A lifecycle configuration (LCC) provides shell scripts that run only when you create the notebook instance or whenever you start one. When you create a notebook instance, you can create a new LCC or attach an LCC that you already have. Lifecycle configuration scripts are useful for the following use cases:
- To run in local mode, as no internet access
- Pull the (TensorFlow, PyTorch , or MXNet) Docker container into a local machine and execute the pip install -U sagemaker command to make use of the Amazon SageMaker Python SDK for code testing.
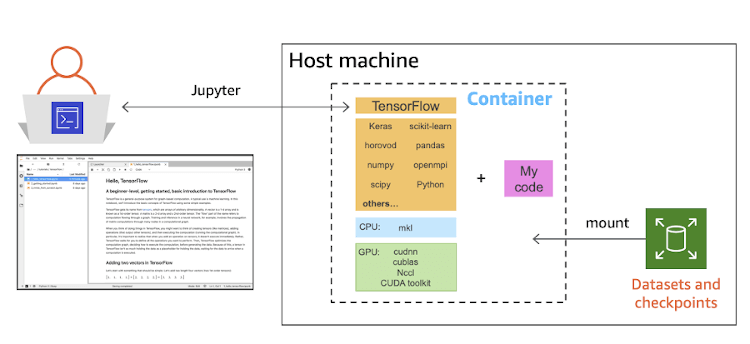
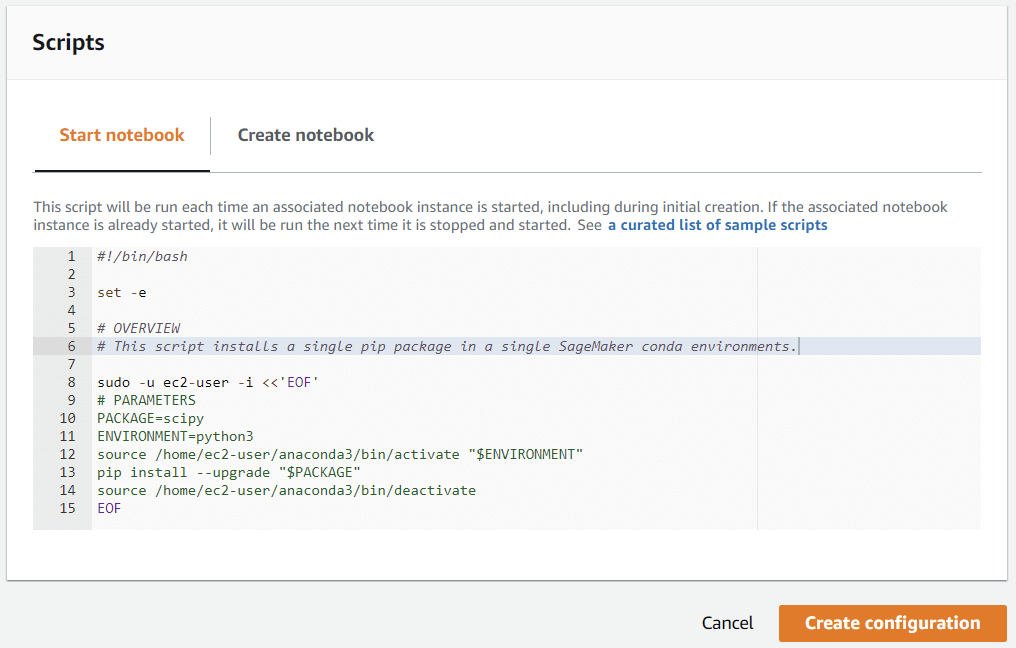
SageMaker Studio
- Visual IDE for machine learning
- SageMaker Notebooks – Jupyter notebooks
- SageMaker Experiments – Organize, capture, compare, and search your ML jobs
- use to automatically create ML experiments by using different combinations of data, algorithms, and parameters
- allows the engineer to automatically track each model’s run, hyperparameters, and results, making it easier to evaluate multiple algorithms and choose the best-performing model
- SageMaker Edge Manager (EOL on 2024)
- Software agent for edge devices
- Model optimized with SageMaker Neo
- Collects and samples data for monitoring, labeling, retraining
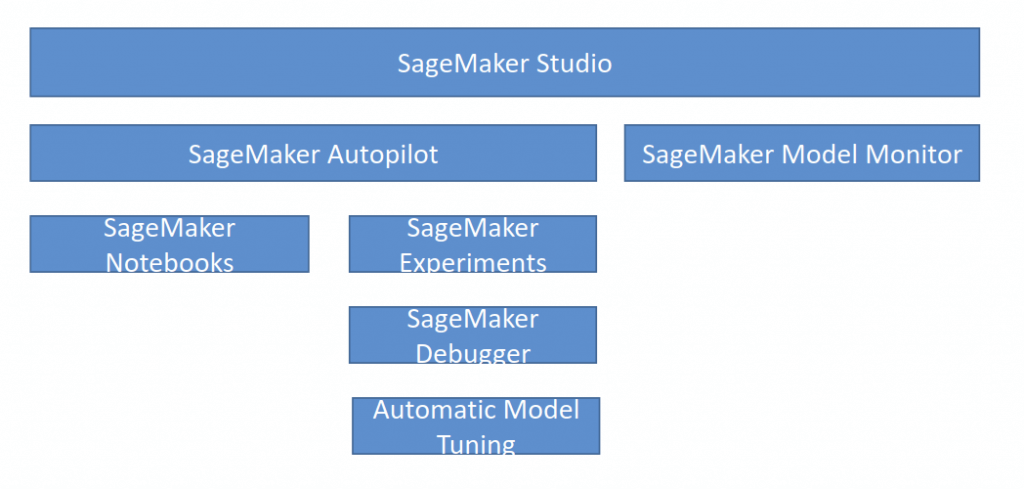
SageMaker and Spark
- Pre-process data as normal with Spark
- Generate DataFrames
- Use sagemaker-spark library
- SageMakerEstimator
- KMeans, PCA, XGBoost
- SageMakerModel
- Notebooks can use the SparkMagic (PySpark) kernel
- Connect notebook to a remote EMR cluster running Spark (or use Zeppelin)
- Training dataframe should have:
- A features column that is a vector of Doubles
- An optional labels column of Doubles
- Call fit on your SageMakerEstimator to get a SageMakerModel
- Call transform on the SageMakerModel to make inferences
- Works with Spark Pipelines as well.
SageMaker on the Edge
- SageMaker Neo
- Train once, run anywhere
- so it’s for “enhances models’ performance after training”
- used to optimize machine learning models for inference on different hardware platforms, including edge devices
- Edge devices
- ARM, Intel, Nvidia processors
- Optimizes code for specific devices
- Tensorflow, MXNet, PyTorch, ONNX, XGBoost, DarkNet, Keras
- Consists of a compiler and a runtime
- models are compiled into an optimized binary, allowing them to run with significantly lower latency and reduced compute resources, making it ideal for applications requiring fast decision-making, such as object detections.
- Train once, run anywhere
- Neo + AWS IoT Greengrass
- Neo-compiled models can be deployed to an HTTPS endpoint
- Hosted on C5, M5, M4, P3, or P2 instances
- Must be same instance type used for compilation
- OR! You can deploy to IoT Greengrass
- This is how you get the model to an actual edge device
- Inference at the edge with local data, using model trained in the cloud
- Uses Lambda inference applications
- Neo-compiled models can be deployed to an HTTPS endpoint
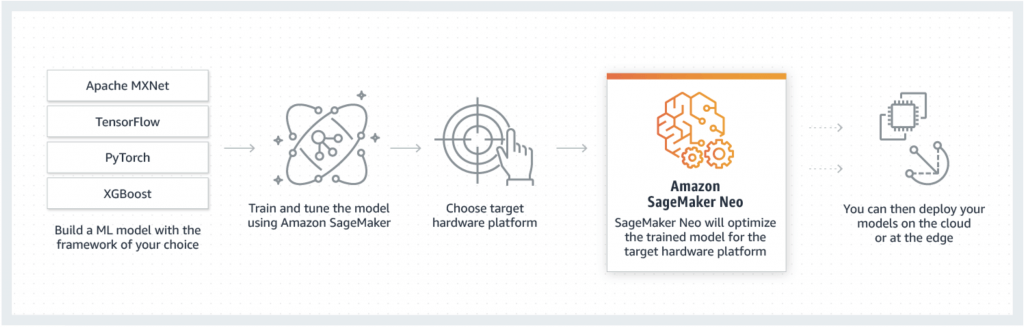
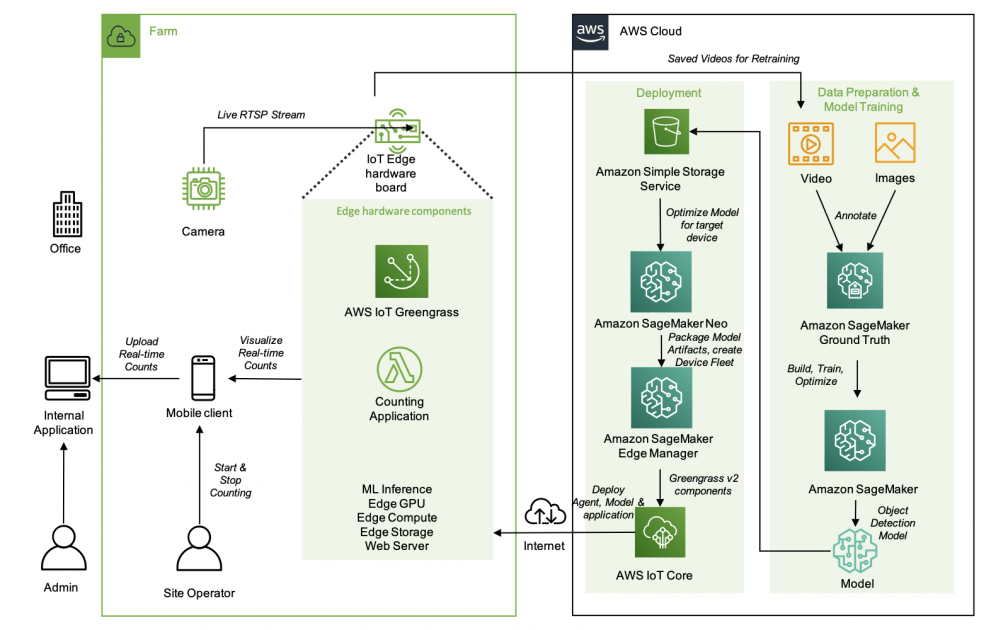
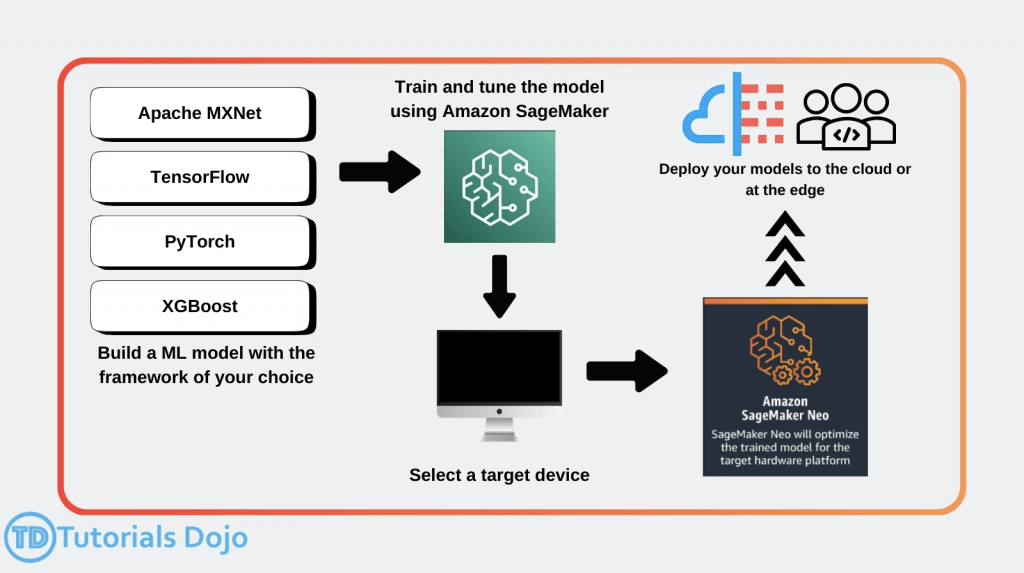
Automate key machine learning tasks and use no-code or low-code solutions
- SageMaker Canvas
- No-code machine learning for business analysts
- Capabilities for tasks
- such as data preparation, feature engineering, algorithm selection, training and tuning, inference, and more.
- Upload csv data (csv only for now), select a column to predict, build it, and make predictions
- Can also join datasets
- Classification or regression
- Automatic data cleaning
- Missing values
- Outliers
- Duplicates
- Share models & datasets with SageMaker Studio
- Custom models: for numeric prediction, categories prediction, and time series forecasting
- Ready-to-use models
- Sentiment analysis
- Entities extraction
- Language detection
- Personal information detection
- Document analysis
- Document queries
- Object detection in images
- Text detection in images
- Expense analysis
- Identity document analysis
- The Finer Points
- Local file uploading must be configured “by your IT administrator.”
- Set up an S3 bucket with appropriate CORS permissions
- Can integrate with Okta SSO
- Canvas lives within a SageMaker Domain that must be manually updated
- Import from Redshift can be set up
- Time series forecasting must be enabled via IAM
- Can run within a VPC
- Local file uploading must be configured “by your IT administrator.”
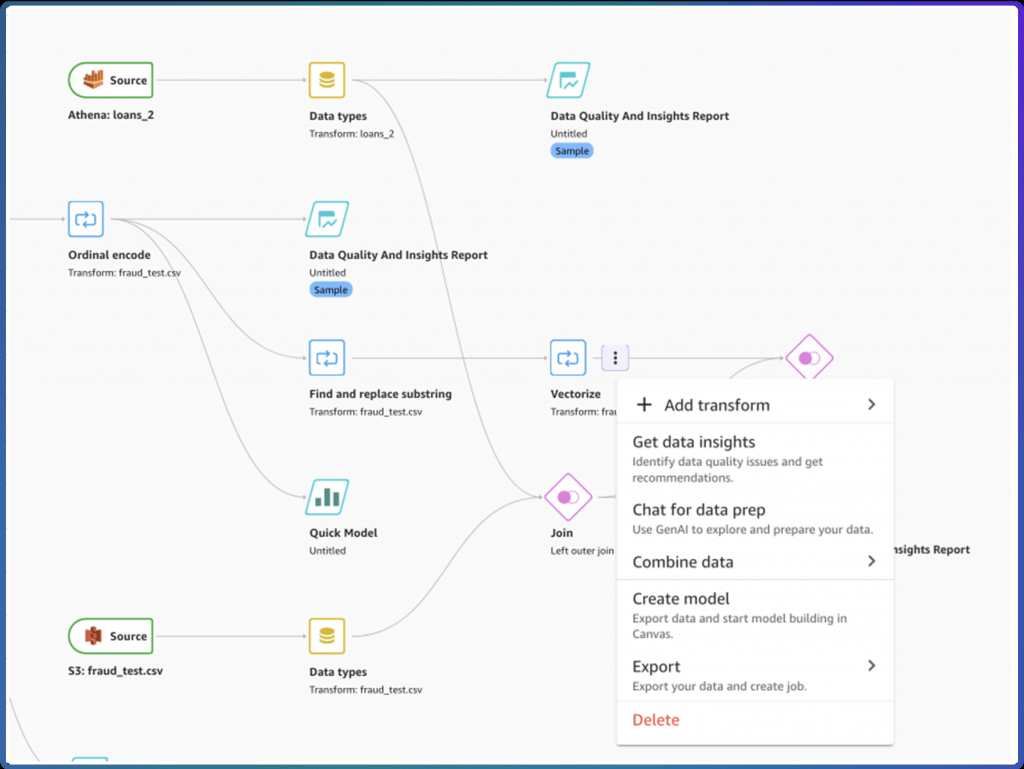
- SageMaker Autopilot (has been integrated into Canvas)
- Automates:
- Algorithm selection
- Data preprocessing
- Model tuning
- All infrastructure
- It does all the trial & error for you
- More broadly this is called AutoML
- Wokflow
- Load data from S3 for training
- Select your target column for prediction
- Automatic model creation
- Model notebook is available for visibility & control
- Model leaderboard
- Ranked list of recommended models
- You can pick one
- Deploy & monitor the model, refine via notebook if needed
- Can add in human guidance
- With or without code in SageMaker Studio or AWS SDK’s
- Problem types:
- Binary classification
- Multiclass classification
- Regression
- Algorithm Types:
- Linear Learner
- XGBoost
- Deep Learning (MLP’s)
- Ensemble mode
- Data must be tabular CSV or Parquet
- Training Modes ???
- HPO (Hyperparameter optimization)
- Ensembling
- Auto
- Explainability ???
- Integrates with SageMaker Clarify
- Transparency on how models arrive at predictions
- Feature attribution
- Uses SHAP Baselines / Shapley Values
- Research from cooperative game theory
- Assigns each feature an importance value for a given prediction
- Automates:
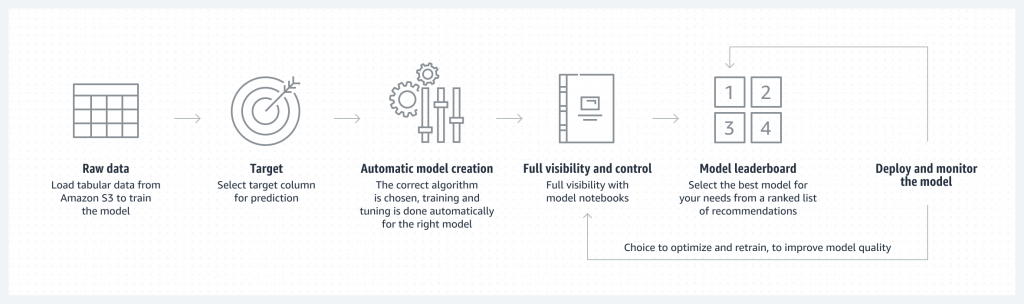
- SageMaker JumpStart
- One-click models and algorithms from model zoos
- provides pre-built models and end-to-end solutions for common machine learning use cases
- Over 150 open source models in NLP, object detections, image classification, etc.
- also provides solution templates that set up infrastructure for common use cases, and executable example notebooks for machine learning with SageMaker AI
- NOT allow testing different algorithms and custom training
Amazon SageMaker Model Cards
- document machine learning models
- capture detailed information about each model, including its background, intended use cases, performance metrics, and business context
Amazon SageMaker Model Registry
- primarily used for model versioning and deployment management
- focuses more on model governance and deployment aspects than documentation
Amazon SageMaker Model Dashboard
- a tool primarily used for visualizing and monitoring the performance of machine learning models deployed on SageMaker endpoints.
Amazon SageMaker Inference Recommender
- helps determine the optimal instance type and configuration for deploying a machine learning model based on performance requirements and cost considerations
- How it works:
- Register your model to the model registry
- Benchmark different endpoint configurations
- Collect & visualize metrics to decide on instance types
- Existing models from zoos may have benchmarks already
- Instance Recommendations
- Runs load tests on recommended instance types
- Takes about 45 minutes
- Endpoint Recommendations
- Custom load test
- You specify instances, traffic patterns, latency requirements,
throughput requirements - Takes about 2 hours
Amazon SageMaker Automatic Scaling
- automatically adjusts the number of instances provisioned for a SageMaker endpoint based on the incoming traffic
- set up a scaling policy to define target metrics, min/max capacity, cooldown periods
- Works with CloudWatch
- Also it’s possible to scheduled actions to perform scaling activities at specific times. These actions can be set to scale either once or on a recurring schedule
- Types
- Scheduled
- Target Tracking
- target tracking scaling policies to dynamically increase or decrease capacity based on a target value for a performance metric
- To increase the target value for the latency metric would delay scaling actions until higher latency is observed.
- A smaller target latency value would drive earlier scaling actions as event traffic begins
- Invocation metrics
- Latency metrics
- Utilization metrics
- Step scaling policies
- define a series of thresholds with associated capacity adjustments
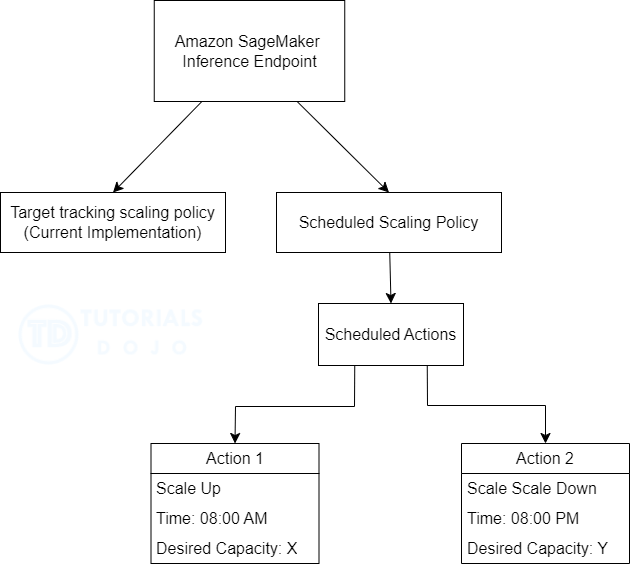
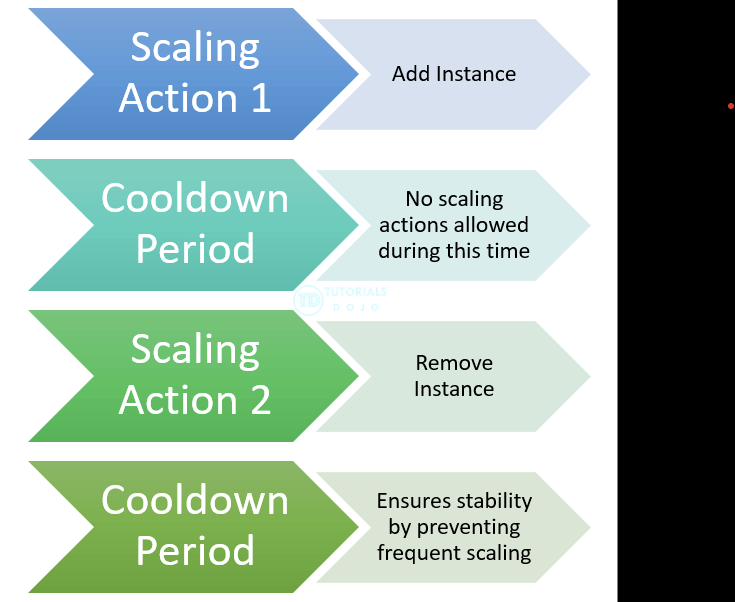
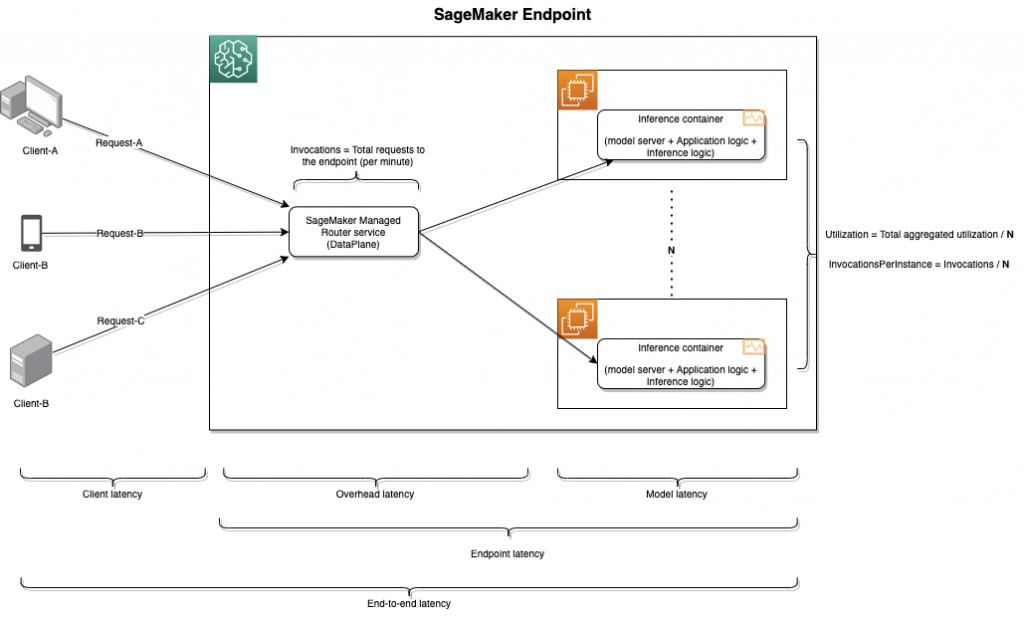
Amazon SageMaker Multi-Model Endpoints
- deploy multiple models behind a single endpoint.
- multiple models that need to be served simultaneously
- Utilize the same set of resources and a shared serving container, which helps reduce hosting costs and deployment overhead. Amazon SageMaker manages the loading of models in memory and scales them based on the traffic patterns to your endpoint.
- or perform A/B testing between different models.
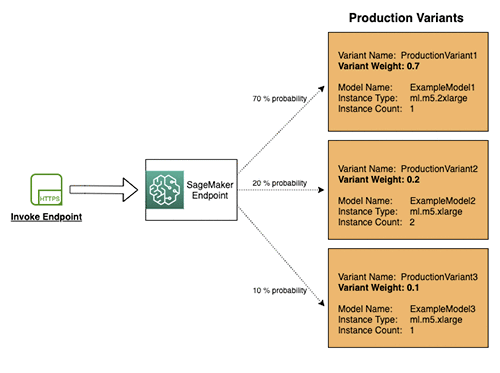
While the benefit of building custom ML models for each use case is higher inference accuracy, the downside is that the cost of deploying models increases significantly, and it becomes difficult to manage so many models in production. These challenges become more pronounced when you don’t access all models at the same time but still need them to be available at all times. Amazon SageMaker multi-model endpoints address these pain points and give businesses a scalable yet cost-effective solution to deploy multiple ML models.
Multi-model endpoints provide a scalable and cost-effective solution to deploying large numbers of models. They use a shared serving container that is enabled to host multiple models. This reduces hosting costs by improving endpoint utilization compared with using single-model endpoints. It also reduces deployment overhead because Amazon SageMaker manages loading models in memory and scaling them based on the traffic patterns to them.
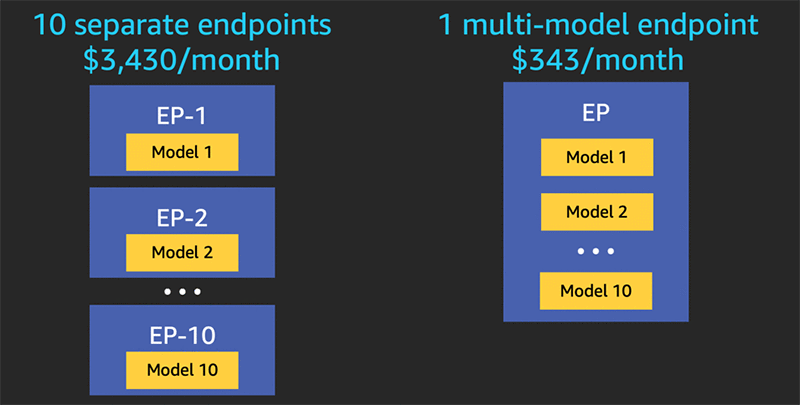
Multi-model endpoints are fully managed and highly available to serve traffic in real-time. You can easily invoke a specific model by specifying the target model name as a parameter in your prediction request.
== OPERATIONS ==
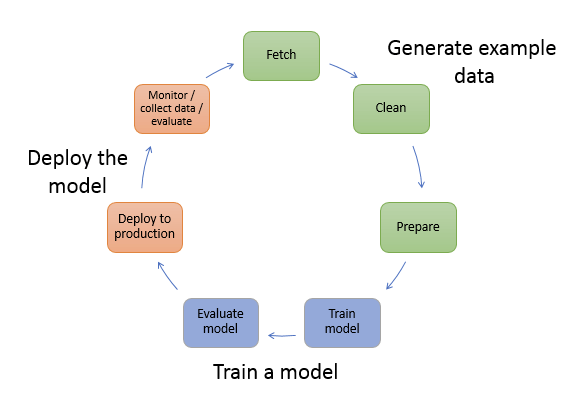
— Prepare Data —
Data prep on SageMaker
- Data Sources
- usually comes from S3
- Ideal format varies with algorithm – often it is RecordIO / Protobuf
- LibSVM is a format for representing data in a simple, text-based, and sparse format, primarily used for support vector machines (SVM).
- RecordIO/Protobuf is a binary data storage format that allows for efficient storage and retrieval of large datasets, commonly used in deep learning and data processing.
- The SageMaker AI Processing job requires specific permissions to access objects in an S3 bucket.
- If the execution role assigned to the job does not have the s3:GetObject permission for the file, it will encounter a 403 Forbidden error when trying to access the file.
- Not “SageMaker Studio”, which primarily is used for tasks within the SageMaker Studio environment.
- Ideal format varies with algorithm – often it is RecordIO / Protobuf
- also ingest from Athena, EMR, Redshift, and Amazon Keyspaces DB
- usually comes from S3
- Apache Spark integrates with SageMaker
- Scikit_learn, numpy, pandas all at your disposal within a notebook
- Processing (example)
- Copy data from S3
- Spin up a processing container
- SageMaker built-in or user provided
- Output processed data to S3
SageMaker Data Wrangler
- Visual interface (in SageMaker Studio) to prepare data for machine learning
- Import data
- Visualize data (Explore and Analysis)
- Transform data (300+ transformations to choose from)
- Or integrate your own custom xforms with pandas, PySpark, PySpark SQL
- Balance Data – create better models for binary classification; for example, detects fraudulent
- Random oversampling
- Random undersampling
- Synthetic Minority Oversampling Technique (SMOTE)
- creates synthetic fraudulent cases and can help address the low number of fraudulent transactions
- Oversampling by using bootstrapping (useless)
- create additional samples by resampling with replacements from the existing minority class
- does not add new data points; ie does not provide enrichment
- Undersampling (not recommended)
- reduce the number of examples from the majority class to the dataset
- drops some data points; ie you risk the loss of potentially valuable information from the majority class.
- Reduce Dimensionality within a Dataset
- Principal Component Analysis (PCA)
- Encode Categorical – encode categorical data that is in string format into arrays of integers
- Ordinal Encode
- One-Hot Encode
- Featurize Text ??
- Character statistics
- Vectorize
- Handle Outliers
- Robust Standard Deviation Numeric Outliers – (Q1/Q3 + n x Standard Deviation)
- Standard Deviation Numeric Outliers – only n x Standard Deviation
- Quantile Numeric Outliers – only Q1/Q3
- Min-Max Numeric Outliers – set upper and lower threshold
- Replace Rare – set a single threshold
- Handle Missing Values
- Fill missing (predefined value)
- Impute missing (mean or median, or most frequent value for categorical data)
- Drop missing (row)
- Process Numeric
- Standard Scaler
- Robust Scaler
- removes the median and scales the data using the interquartile range (IQR), making it less vulnerable to outliers than the Standard Scaler or Min-Max Scaler
- Min Max Scaler
- Max Absolute Scaler
- L2 Normalization
- Z-Score Normalization
- Split Data
- Randomized
- Ordered: best for time-sensitive data, like sorted by date
- Stratified: ensure that each split represents the overall dataset proportionally across a specified key, such as class labels in classification tasks, ie, “all categories of data are represented in each set”.
- Key-Based
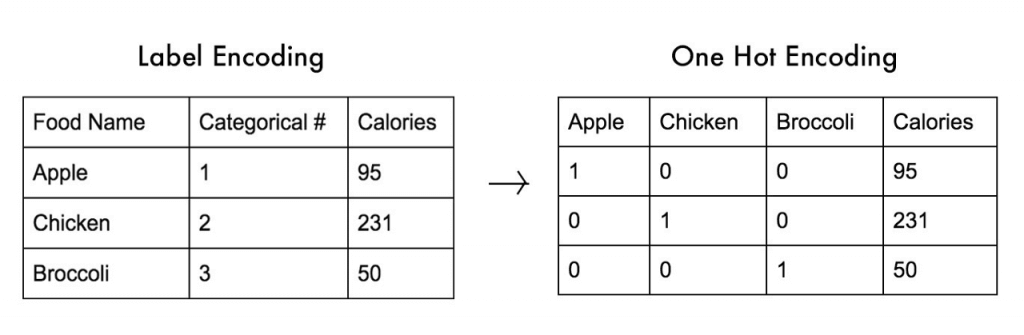
Aspect | StandardScaler | Normalizer |
---|---|---|
Operation Basis | Feature-wise (across columns) | Sample-wise (across rows) |
Purpose | Standardizes features to zero mean and unit variance | Scales samples to unit norm (L2 by default) |
Impact on Data | Alters the mean and variance of each feature | Adjusts the magnitude of each sample vector |
Common Use Cases | Regression, PCA, algorithms sensitive to variance | Text classification, k-NN, direction-focused tasks |
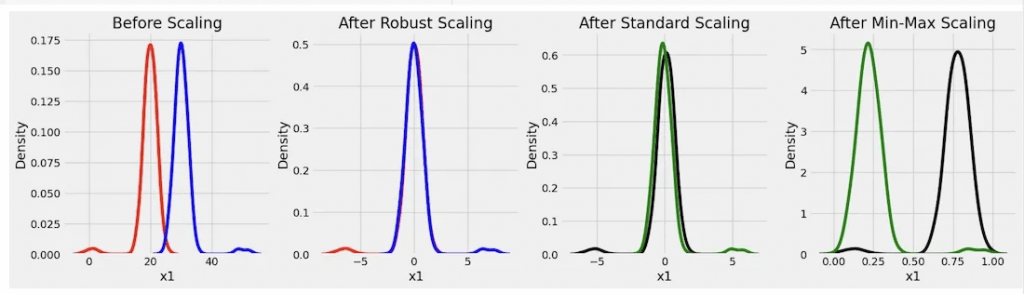
- preprocessing large text datasets (super on NLP tasks)
- handle various data formats
- supports common NLP preprocessing techniques
- tokenization
- stemming
- stop word removal
- “Quick Model” to train your model with your data and measure its results
- Troubleshooting
- Make sure your Studio user has appropriate IAM roles
- Make sure permissions on your data sources allow Data Wrangler access
- Add AmazonSageMakerFullAccess policy
- EC2 instance limit
- If you get “The following instance type is not available…” errors
- May need to request a quota increase
- Service Quotas / Amazon SageMaker / Studio KernelGateway Apps running on ml.m5.4xlarge instance
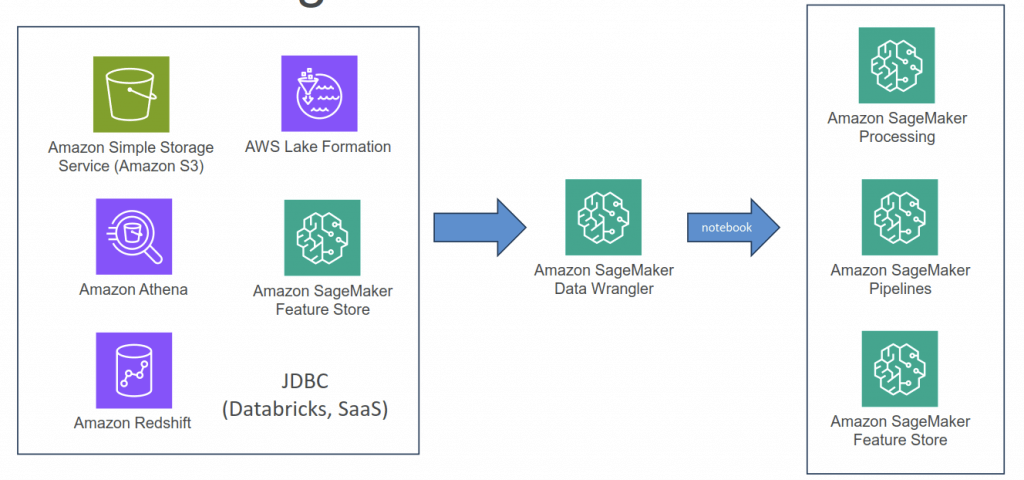
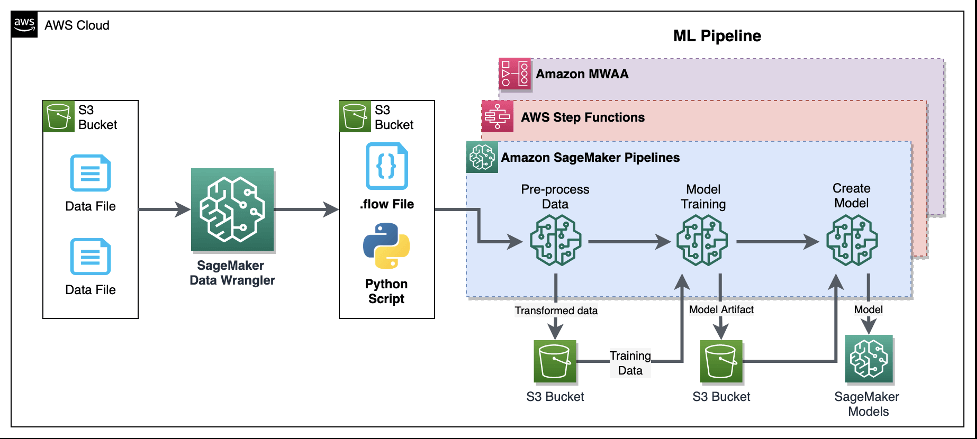
— Processing Job —
Amazon SageMaker Processing
- a fully managed service that simplifies the process of running data processing and model evaluation jobs
- run data pre and post processing, feature engineering, and model evaluation tasks on SageMaker
- built-in support for TensorFlow and Hugging Face’s Transformers and can directly interact with data stored in Amazon S3
- Input modes
- File mode
- source: S3 Bucket
- File mode downloads training data to a local directory in a Docker container.
- Fast file mode
- source: S3 Bucket
- At the start of training, fast file mode identifies the data files but does not download them. Training can start without waiting for the entire dataset to download.
- Fast file mode doesn’t support augmented manifest files.
- This means that your dataset no longer needs to fit into the training instance storage space as a whole, and you don’t need to wait for the dataset to be downloaded to the training instance before training starts.
- Pipe mode
- source: S3 Bucket
- Streaming can provide faster start times and better throughput than file mode.
- When you stream the data directly, you can reduce the size of the Amazon EBS volumes used by the training instance. Pipe mode needs only enough disk space to store the final model artifacts.
- data is pre-fetched from Amazon S3 at high concurrency and throughput, and streamed into a named pipe, which also known as a First-In-First-Out (FIFO) pipe for its behavior.
- can use the optimized protobuf recordIO data format for faster.
- Amazon S3 Express One Zone
- source: S3 Bucket
- high-performance, single Availability Zone storage class that can deliver consistent, single-digit millisecond data access for the most latency-sensitive applications
- Amazon FSx for Lustre
- source: FSx for Lustre
- scale to hundreds of gigabytes of throughput and millions of IOPS with low-latency file retrieval.
- Amazon EFS
- source: EFS
- File mode
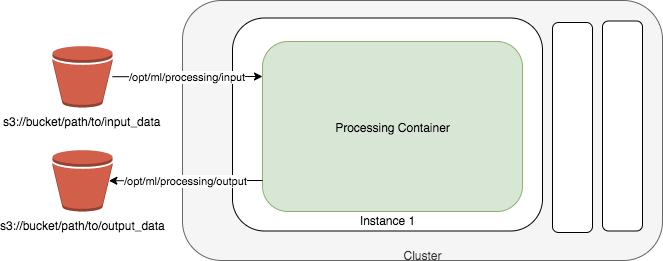
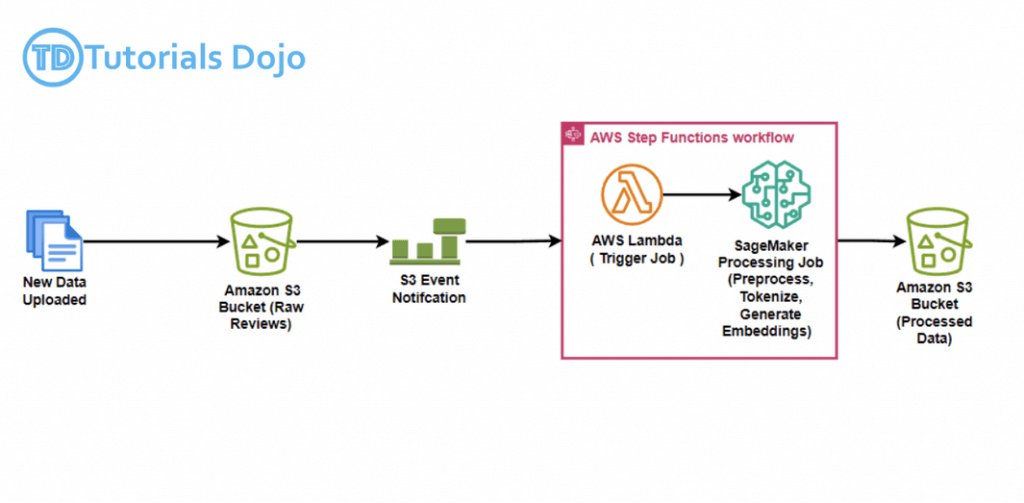
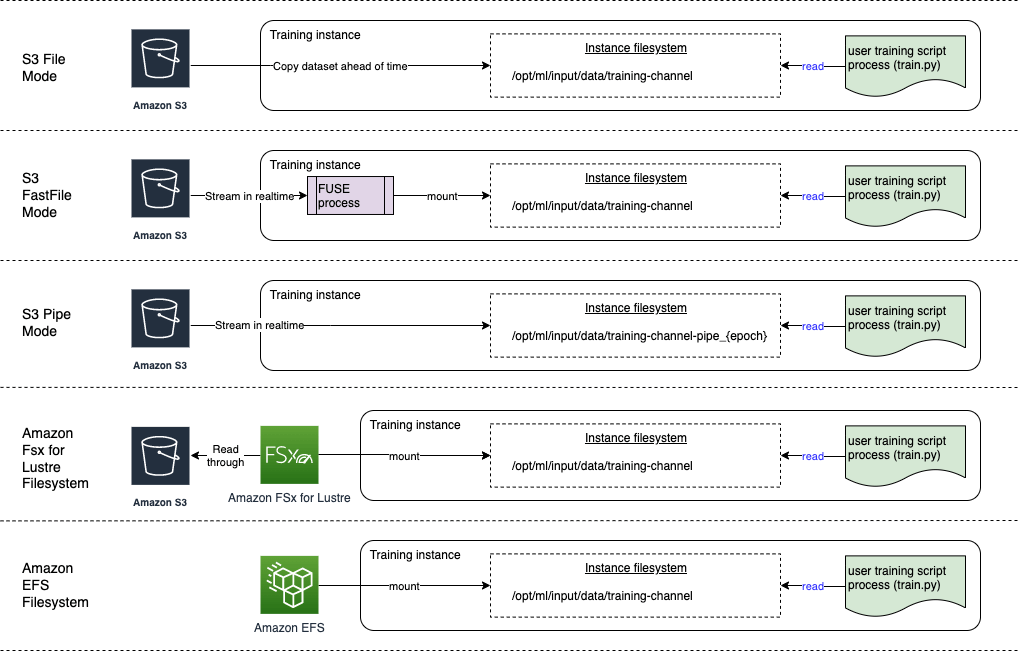
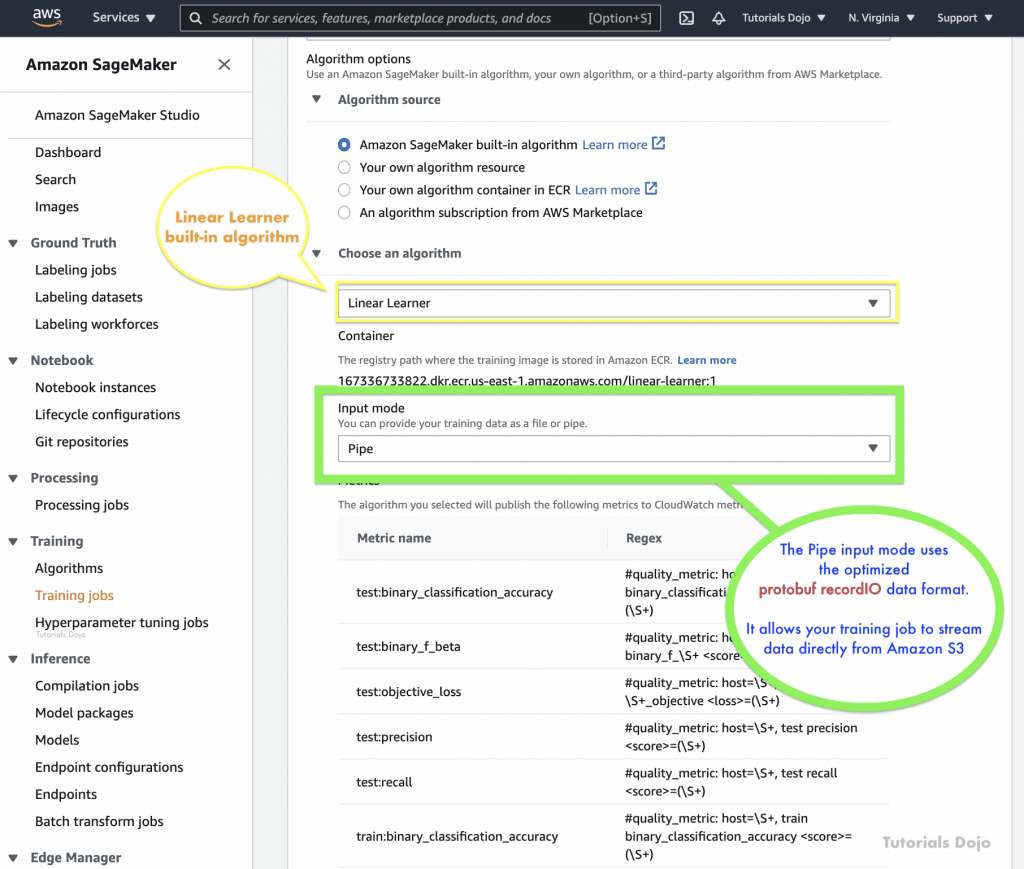
— Feature Creation & Store —
SageMaker Feature Store
- A “feature” is just a property used to train a machine learning model.
- Like, you might predict someone’s political party based on “features” such as their address, income, age, etc.
- Machine learning models require fast, secure access to feature data for training.
- keep it organized and share features across different models
- Find, discover, and share features in Studio
- also allows for cross-account access
- Store modes
- Online stores are typically used for low-latency access during inference
- Offline stores used for training or batch inference
- Features organized into Feature Groups
- the primary resource within Feature Store that holds both the data and metadata used for training or making predictions with an ML model
- A feature group represents a logical collection of features that describe individual records
- Data Ingestion
- STREAMING access via PutRecord / GetRecord API’s
- BATCH access via the offline S3 store (use with anything that hits S3, like Athena, Data Wrangler. Automatically creates a Glue Data Catalog for you.)
- Security
- Encrypted at rest and in transit
- Works with KMS customer master keys
- Fine-grained access control with IAM
- May also be secured with AWS PrivateLink
- Steps
- Setup new feature store
- Define a new feature group
- organizes the features and metadata for model training and inference
- Connect with an offline store to the feature group
- an offline store, which stores historical data in Amazon S3 and supports batch processing
- Load data into the offline store using batch processing
- Define a new feature group
- Add/Update new feature into existing groups
- Utilize the UpdateFeatureGroup command to incorporate the new feature into the feature group. Specify the attribute name and type.
- Apply the PutRecord command to ingest or overwrite records, ensuring that the newly added feature has data populated across both historical and new records
- update the records that are missing data for the new attribute.
- Setup new feature store
- Create an endpoint for feature queries is for querying during inference
- Schedule regular feature updates also is for maintenance, not for new store establishment
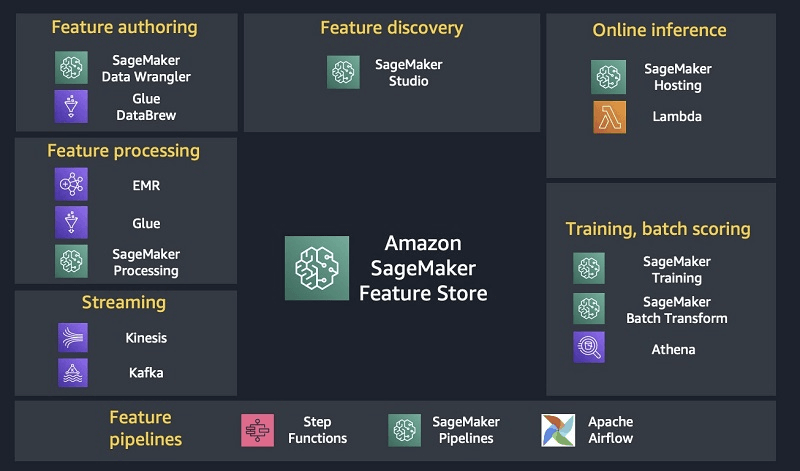
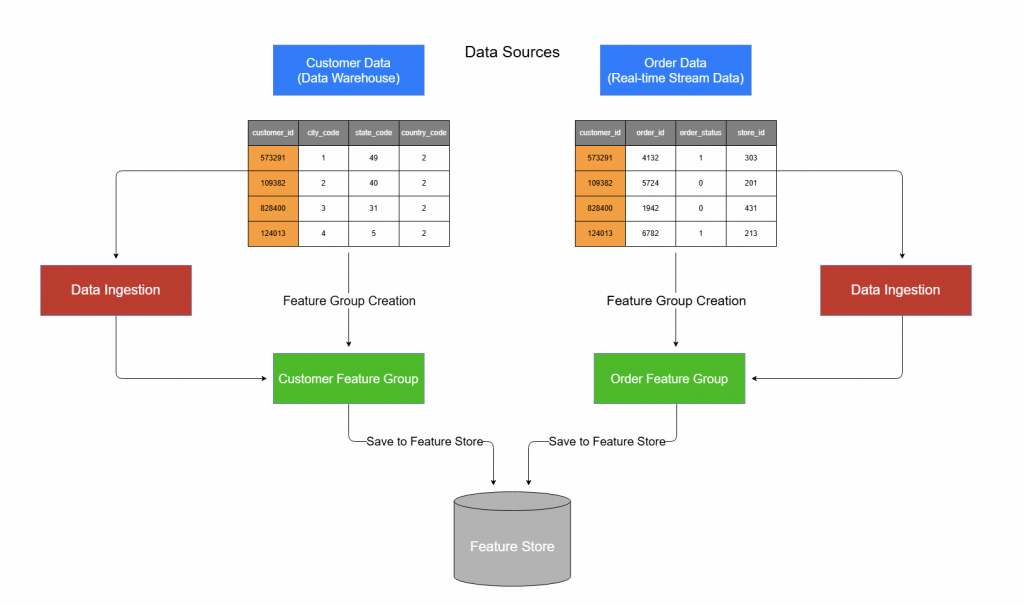
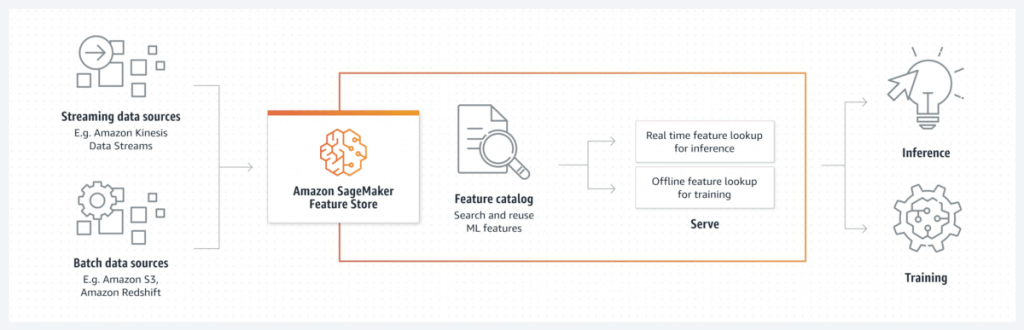
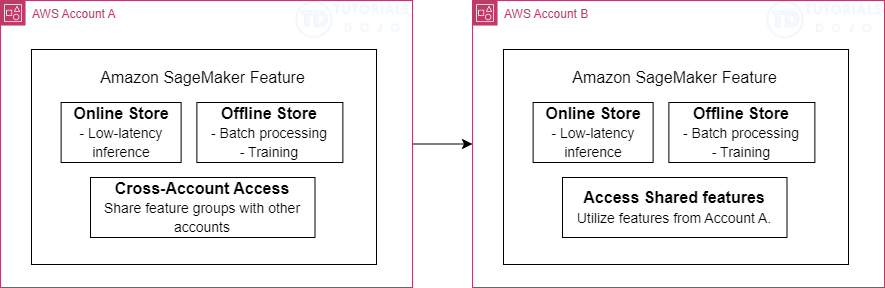
— Model Training —
Training/Deployment on SageMaker
- Create a training job
- URL of S3 bucket with training data
- ML compute resources
- URL of S3 bucket for output
- ECR path to training code
- Training options
- Built-in training algorithms
- Spark MLLib
- Custom Python Tensorflow / MXNet code
- PyTorch, Scikit-Learn, RLEstimator
- XGBoost, Hugging Face, Chainer
- …
- Save your trained model to S3
- Can deploy two ways:
- Persistent endpoint for making individual predictions on demand
- SageMaker Batch Transform to get predictions for an entire dataset
- Lots of cool options
- Inference Pipelines for more complex processing
- SageMaker Neo for deploying to edge devices
- Elastic Inference for accelerating deep learning models
- Automatic scaling (increase # of endpoints as needed)
- Shadow Testing evaluates new models again
- Incremental Training
- use the artifacts from existing model and use an expanded dataset
- use cases
- train a new model with an expanded dataset that contains an underlying pattern
- use a portain of the model artifacts
- resume a stopped training job
- train several variants of model
Amazon SageMaker Automatic Model Tuning (AMT)
- hyperparameter optimization of the model
- “HyperParameter Tuning Job” that trains as many combinations as you’ll allow
- It learns as it goes
- Best Practices
- Don’t optimize too many hyperparameters at once
- Limit your ranges to as small a range as possible
- Use logarithmic scales when appropriate
- Don’t run too many training jobs concurrently
- This limits how well the process can learn as it goes
- Make sure training jobs running on multiple instances report the correct objective metric in the end
- Strategies
- Grid Search: chooses combinations of values from the range of categorical values that you specify. Only categorical parameters are supported when using the grid search strategy
- Random Search
- Bayesian optimization: treats as regression problem
- Stop the training jobs that a hyperparameter tuning job launches early when they are not improving significantly as measured by the objective metric. Stopping training jobs early can help reduce compute time and helps you avoid overfitting your model.
- use early stopping to compare the current objective metric (accuracy) against the median of the running average of the objective metric
- Use warm start to start a hyperparameter tuning job using one or more previous tuning jobs as a starting point. The results of previous tuning jobs are used to inform which combinations of hyperparameters to search over in the new tuning job.
- Reasons
- To gradually increase the number of training jobs over several tuning jobs based on results after each iteration.
- To tune a model using new data that you received.
- To change hyperparameter ranges that you used in a previous tuning job, change static hyperparameters to tunable, or change tunable hyperparameters to static values.
- Types
- IDENTICAL_DATA_AND_ALGORITHM
- uses the same input data and training image as the parent tuning jobs
- use the same training data as you used in a previous hyperparameter tuning job, but you want to increase the total number of training jobs or change ranges or values of hyperparameters
- TRANSFER_LEARNING
- use different input data, hyperparameter ranges, and other hyperparameter tuning job parameters than the parent tuning jobs
- IDENTICAL_DATA_AND_ALGORITHM
- Reasons
SageMaker Training Compiler
- Integrated into AWS Deep Learning Containers (DLCs)
- Can’t bring your own container
- Compile & optimize training jobs on GPU instances
- Can accelerate training up to 50%
- Converts models into hardware-optimized instructions
- Tested with Hugging Face transformers library, or bring your own model
- Incompatible with SageMaker distributed training libraries
- Best practices:
- Ensure GPU instances are used (ml.p3, ml.p4)
- PyTorch models must use PyTorch/XLA’s model save function
- Enable debug flag in compiler_config parameter to enable debugging
— Deploy Inference Model —
Inference Model Deployment
- getting predictions, or inferences, from your trained machine learning models
- approaches
- Deploy a machine learning model in a low-code or no-code environment
- deploy pre-trained models using Amazon SageMaker JumpStart through the Amazon SageMaker Studio interface
- Use code to deploy machine learning models with more flexibility and control
- deploy their own models with customized settings for their application needs using the ModelBuilder class in the SageMaker AI Python SDK, which provides fine-grained control over various settings, such as instance types, network isolation, and resource allocation.
- Deploy machine learning models at scale
- use the AWS SDK for Python (Boto3) and AWS CloudFormation along with your desired Infrastructure as Code (IaC) and CI/CD tools
- Deploying a model to an endpoint
- ProductVariant for each model that you want to deploy
- VariantWeight to specify how much traffic you want to allocate to each model
- Cost optimization
- Model performance optimization with SageMaker Neo.
- automatically optimizing models to run in environments like AWS Inferentia chips.
- Automatic scaling of Amazon SageMaker AI models.
- Use autoscaling to dynamically adjust the compute resources for your endpoints based on incoming traffic patterns, which helps you optimize costs by only paying for the resources you’re using at a given time.
- Model performance optimization with SageMaker Neo.
- Deploy a machine learning model in a low-code or no-code environment
- Inference Modes/Endpoint Type (deploying the model to an endpoint)
- Real-time inference
- inference workloads where you have real-time, interactive, low latency requirements
- short processing time (up to 60 seconds)
- (On-demand) Serverless inference
- workloads which have idle periods between traffic spurts and can tolerate cold starts
- without configuring or managing any of the underlying infrastructure
- but, cannot configure a VPC for the endpoint
- also only support short processing time (up to 60 seconds)
- the memory requirements fit within the 6 GB memory and 200 maximum concurrency limits of serverless endpoints
- With “provisioned concurrency”, you can mitigate cold starts and get predictable
- keep the endpoints warm and ready to respond to requests instantaneously
- but it would cost
- performance characteristics for their workloads
- Asynchronous inference
- queues incoming requests and processes them asynchronously
- requests with large payload sizes (up to 1GB), long processing times (up to 60 minutes), and near real-time latency requirements
- Real-time inference
- Batch transform
- Preprocess datasets to remove noise or bias that interferes with training or inference from your dataset.
- Get inferences from large datasets (minimum 100MB per dataset).
- Run inference when you don’t need a persistent endpoint.
- appropriate for workloads that do not need to return an inference for each request to the model
- Associate input records with inferences to help with the interpretation of results.
- suitable for long-term monitoring and trend analysis
- especially when the task can be scheduled and does not require immediate real-time responses
- can handle processing jobs that take anywhere from a few minutes to several hours or even days, making it suitable for long-running jobs like daily sales predictions.
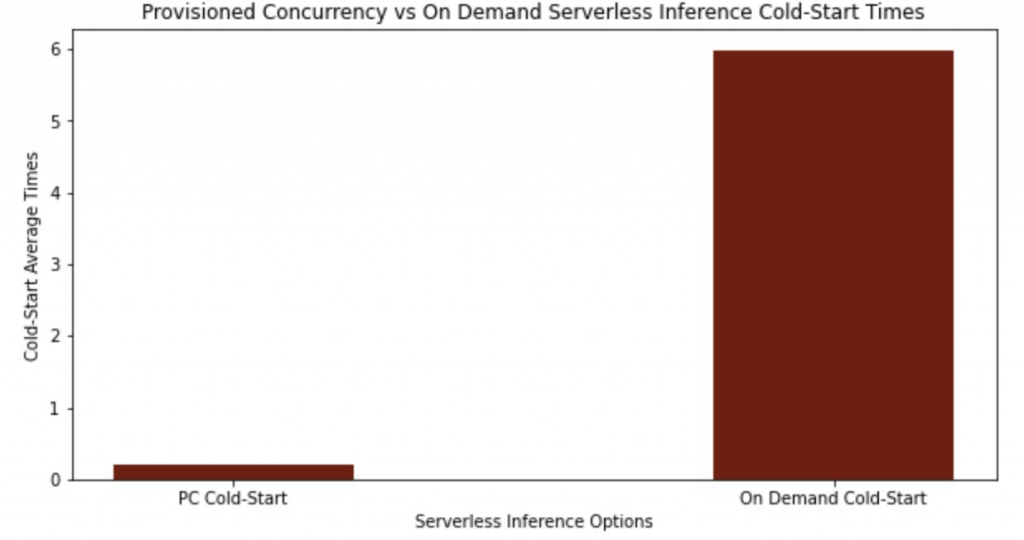
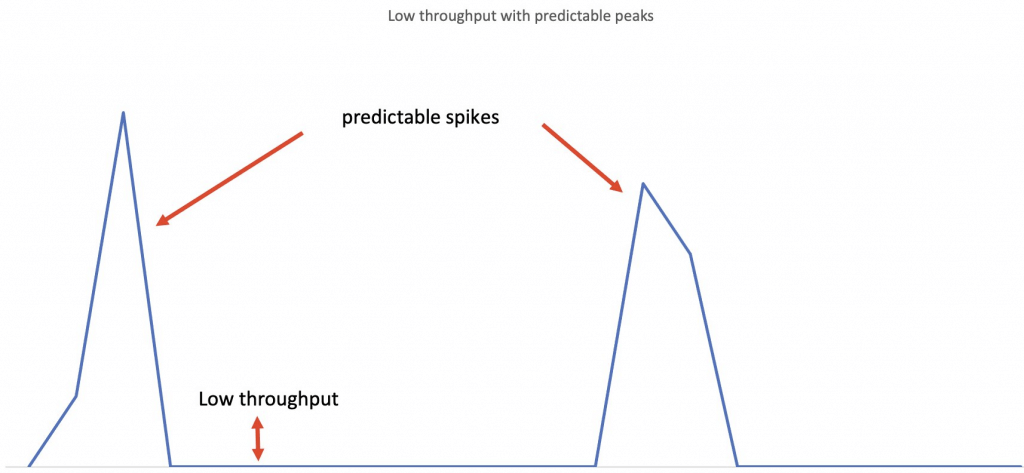
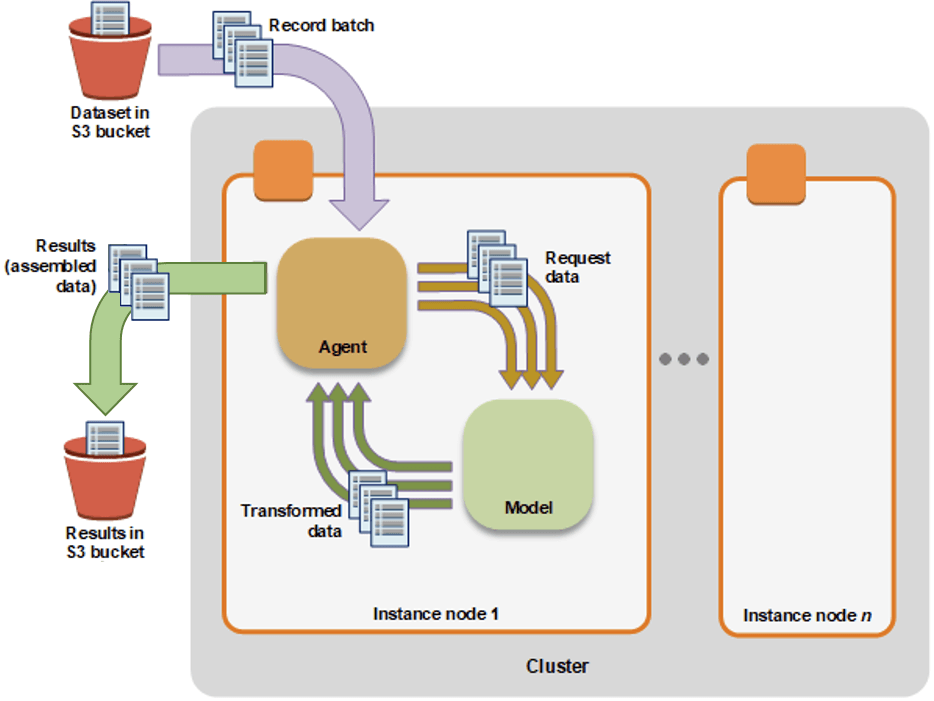
- Deployment Safeguards
- Deployment Guardrails
- For asynchronous or real-time inference endpoints
- Controls shifting traffic to new models
- Blue/Green Deployments
- ie “All at once”: shift everything, monitor, terminate blue fleet
- Canary
- allows you to deploy new versions of machine learning models or applications to a small subset of users or traffic
- Linear
- Shift traffic in linearly spaced steps
- does not provide the initial small-scale rollout and evaluation phase that Canary deployment offers
- (no good!) In-place
- update the application by using existing compute resources
- You stop the current version of the application. Then, you install and start the new version of the application
- Auto-rollbacks
- Shadow
- runs the new version alongside/parallelly the old version for testing without affecting live traffic
- Compare performance of shadow variant to production
- particularly valuable when user inference feedback isn’t necessary
- You monitor in SageMaker console and decide when to promote it
- Deployment Guardrails
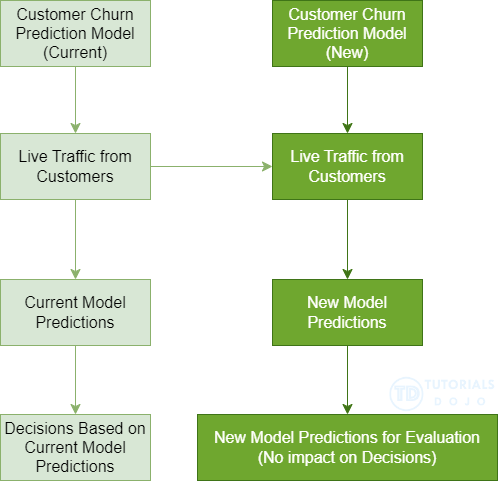
Use case 1 | Use case 2 | Use case 3 | |
---|---|---|---|
SageMaker AI feature | Use JumpStart in Studio to accelerate your foundational model deployment. | Deploy models using ModelBuilder from the SageMaker Python SDK. | Deploy and manage models at scale with AWS CloudFormation. |
Description | Use the Studio UI to deploy pre-trained models from a catalog to pre-configured inference endpoints. This option is ideal for citizen data scientists, or for anyone who wants to deploy a model without configuring complex settings. | Use the ModelBuilder class from the Amazon SageMaker AI Python SDK to deploy your own model and configure deployment settings. This option is ideal for experienced data scientists, or for anyone who has their own model to deploy and requires fine-grained control. | Use AWS CloudFormation and Infrastructure as Code (IaC) for programmatic control and automation for deploying and managing SageMaker AI models. This option is ideal for advanced users who require consistent and repeatable deployments. |
Optimized for | Fast and streamlined deployments of popular open source models | Deploying your own models | Ongoing management of models in production |
Considerations | Lack of customization for container settings and specific application needs | No UI, requires that you’re comfortable developing and maintaining Python code | Requires infrastructure management and organizational resources, and also requires familiarity with the AWS SDK for Python (Boto3) or with AWS CloudFormation templates. |
Recommended environment | A SageMaker AI domain | A Python development environment configured with your AWS credentials and the SageMaker Python SDK installed, or a SageMaker AI IDE such as SageMaker JupyterLab | The AWS CLI, a local development environment, and Infrastructure as Code (IaC) and CI/CD tools |
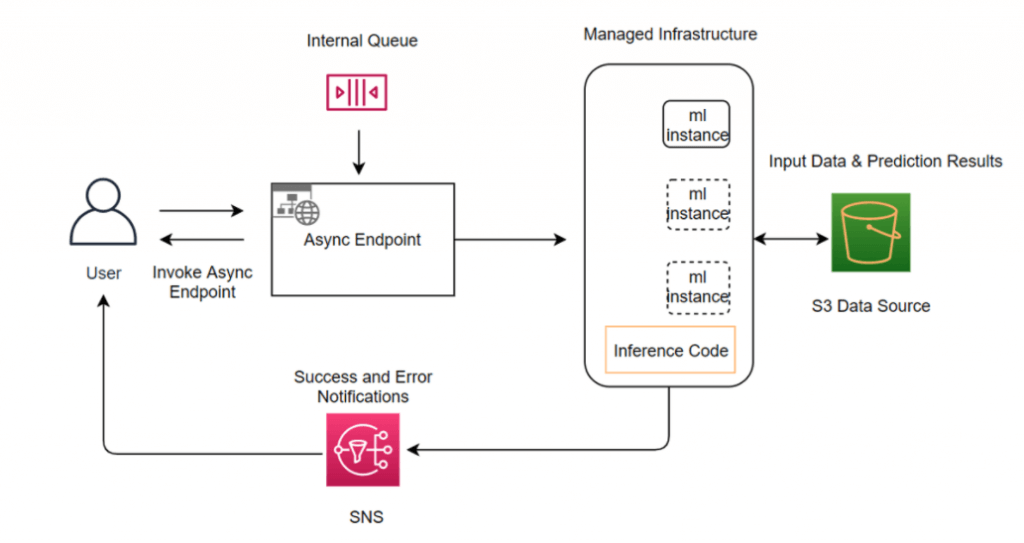
Inference Pipelines
- Linear sequence of 2-15 containers
- Any combination of pre-trained built-in algorithms or your own algorithms in Docker containers
- Combine pre-processing, predictions, post-processing
- Spark ML and scikit-learn containers OK
- Spark ML can be run with Glue or EMR
- Serialized into MLeap format
- Can handle both real-time inference and batch transforms
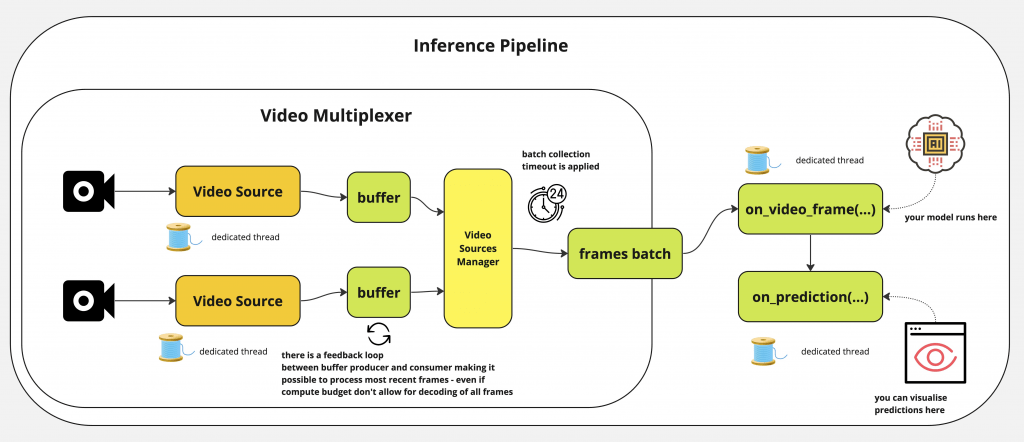
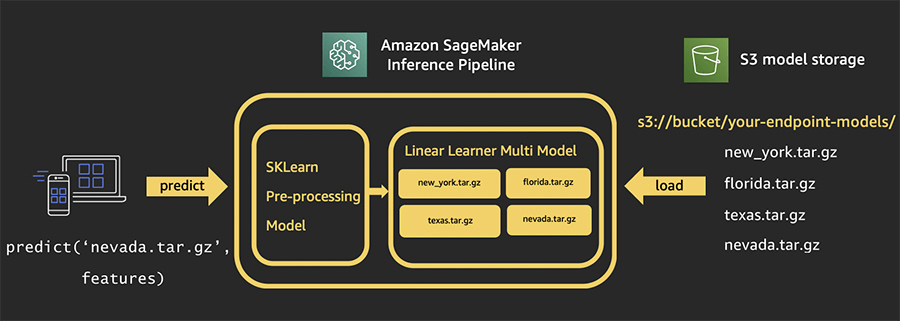
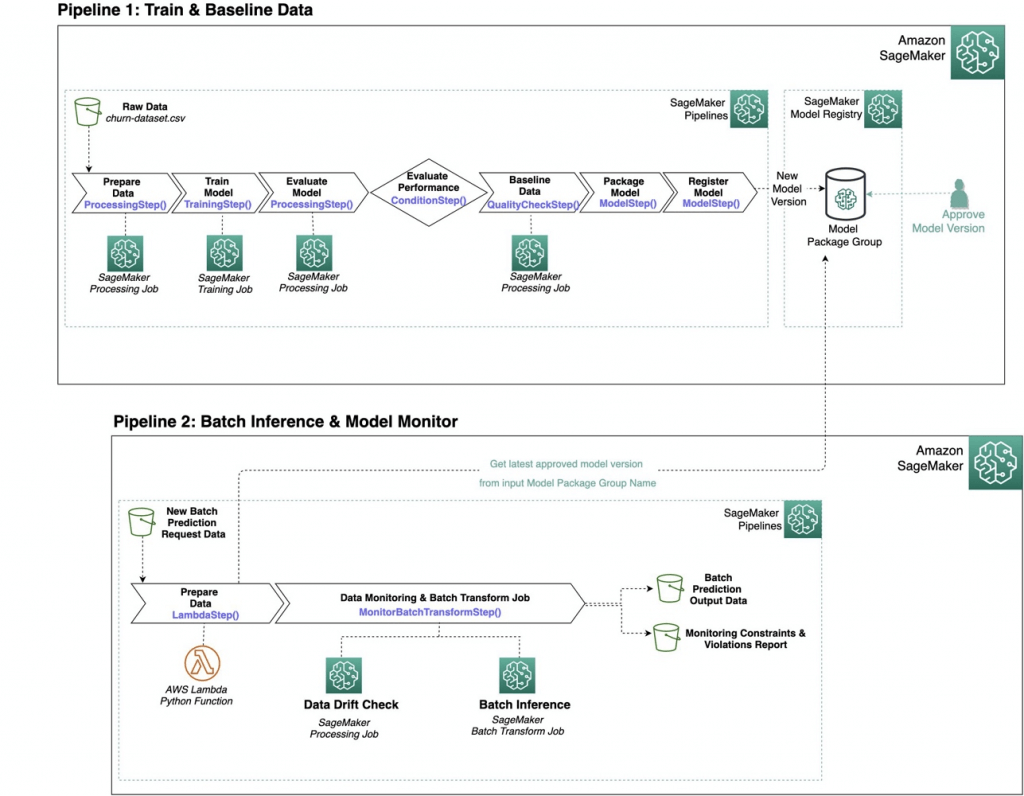
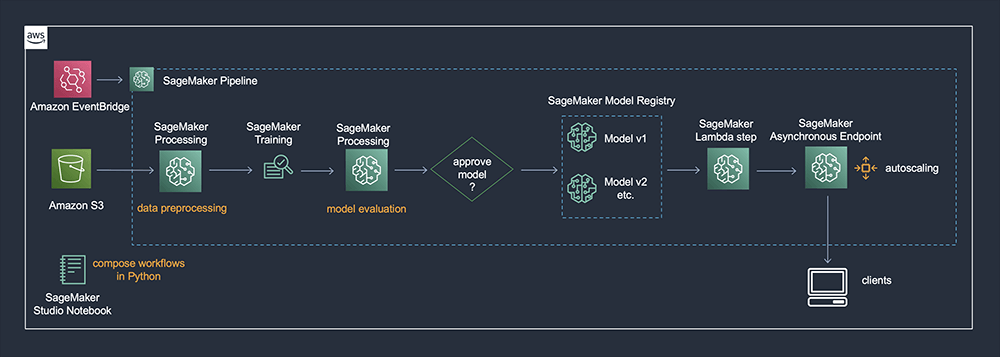
— Quality Monitor —
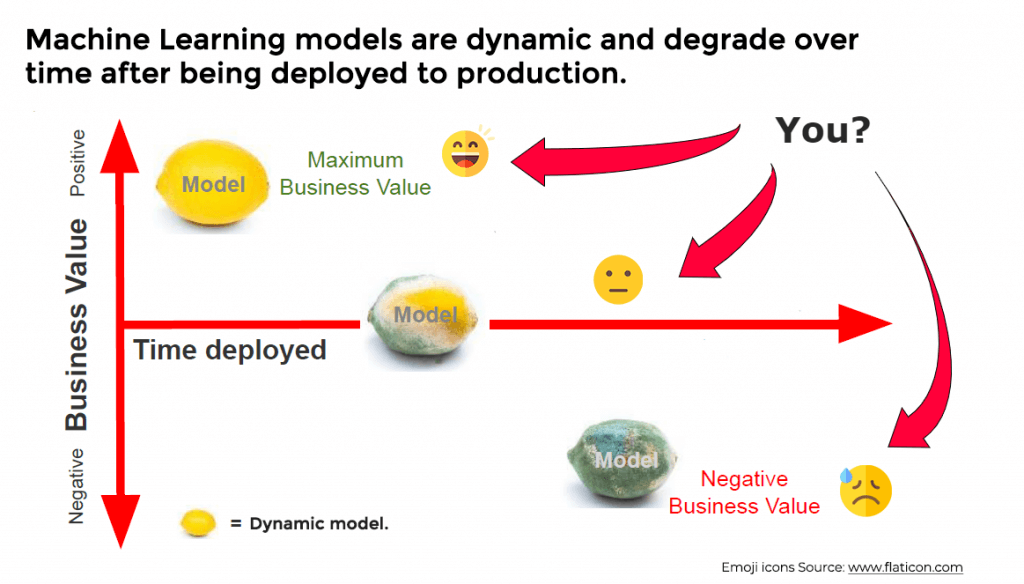
SageMaker Model Monitor
- Data Drift is the phenomenon where the distribution of the input data changes over time, potentially leading to degraded model performance
- Monitors the quality of ML model predictions in production
- Data quality – Monitor drift in data quality.
- the statistical properties of input data change over time, resulting in a decrease in model accuracy.
- for example, changes in patient demographics and conditions reflects a shift in data distribution
- Solution: use Amazon SageMaker Model Monitor, which continuously monitors the data quality of deployed models. This allows data scientists and machine learning engineers to detect drifts in real-time and take corrective actions.
- Use the ModelDataQualityMonitor class to establish a baseline for input data quality. Deploy the baseline to SageMaker Model Monitor to track changes in data patterns and set up notifications in CloudWatch for any detected deviations as primarily used to track the quality of input data and its distribution but does not monitor the importance of features in model predictions
- the statistical properties of input data change over time, resulting in a decrease in model accuracy.
- Model quality – Monitor drift in model quality metrics, such as accuracy.
- Create a performance baseline using the ModelPerformanceMonitor class. Deploy this baseline to SageMaker Model Monitor and configure it to track the model’s prediction accuracy and trigger alerts if any major variations occur as only tracks the overall performance of the model (such as accuracy or precision)
- Bias drift for models in production – Monitor bias in your model’s predictions.
- a predictive model starts to favor specific groups over others because of changes in data distribution or model parameters. This can lead to imbalanced outcomes.
- for example, Disproportionate flagging of certain patient groups indicates bias
- Solution: use Amazon SageMaker Clarify, detect and explain biases in machine learning models. It offers tools to assess and comprehend bias in the data and model predictions, ensuring the model maintains fairness and equality across various demographic groups.
- Create a baseline for monitoring bias using the ModelBiasMonitor class. Deploy this baseline to SageMaker Model Monitor and periodically check for bias drift. Set up Amazon CloudWatch to send alerts when violations are detected; mainly for identifying bias in predictions related to specific attributes such as gender or race, not changes in feature attribution between inputs
- a predictive model starts to favor specific groups over others because of changes in data distribution or model parameters. This can lead to imbalanced outcomes.
- Feature attribution drift for models in production – Monitor drift in feature attribution.
- the significance of various features in a predictive model changes over time. This can happen when the relationships between features and outcomes evolve.
- for example, changing impacts of various health factors
- Solution: use Amazon SageMaker Clarify, assist in understanding feature attribution by offering feature importance scores. This helps data scientists monitor and handle changes in feature importance over time, ensuring that the model continues to generate accurate and reliable predictions.
- The ModelExplainabilityMonitor leverages SHAP (Shapley Additive Explanations) values to track the contribution of each feature. This feature attribution monitoring ensures that if a model begins to emphasize one input (such as driving history) more than another (like age), it can be detected and trigger alerts in Amazon CloudWatch
- the significance of various features in a predictive model changes over time. This can happen when the relationships between features and outcomes evolve.
- (NOT) Virtual Drift , typically refers to a situation where the model’s performance degrades due to operational issues, such as infrastructure or deployment changes.
- Data quality – Monitor drift in data quality.
- Get alerts on quality deviations on your deployed models (via CloudWatch)
- Visualize data drifts
- Detect anomalies & outliers
- Detect new features
- No code needed
- Could “analyze the training data and generate a baseline of statistics and constraints from processed data”
- Integrates with SageMaker Clarify for bias detection and data drift monitoring
- Data is stored in S3 and secured
- Monitoring jobs are scheduled via a Monitoring Schedule
- Metrics are emitted to CloudWatch
- CloudWatch notifications can be used to trigger alarms
- You’d then take corrective action (retrain the model, audit the data)
- Integrates with Tensorboard, QuickSight, Tableau
- Or just visualize within SageMaker Studio
- Data Capture is a feature of SageMaker endpoints
- to record data that you can then use for training, debugging, and monitoring
- Data Capture runs asynchronously without impacting production traffic
- Assume that the model and its endpoint have already been deployed, and we need only to enable Data Capture feature and deploy job definition with a schedule. Data Capture logs are used as input data for the Model Monitor. Data Capture uploads the endpoint inputs and the inference outputs into S3 bucket. Model Monitor automatically parses the captured data and compares metrics with the baseline created for the model.
- Support “real-time endpoints” and “batch-transform jobs”
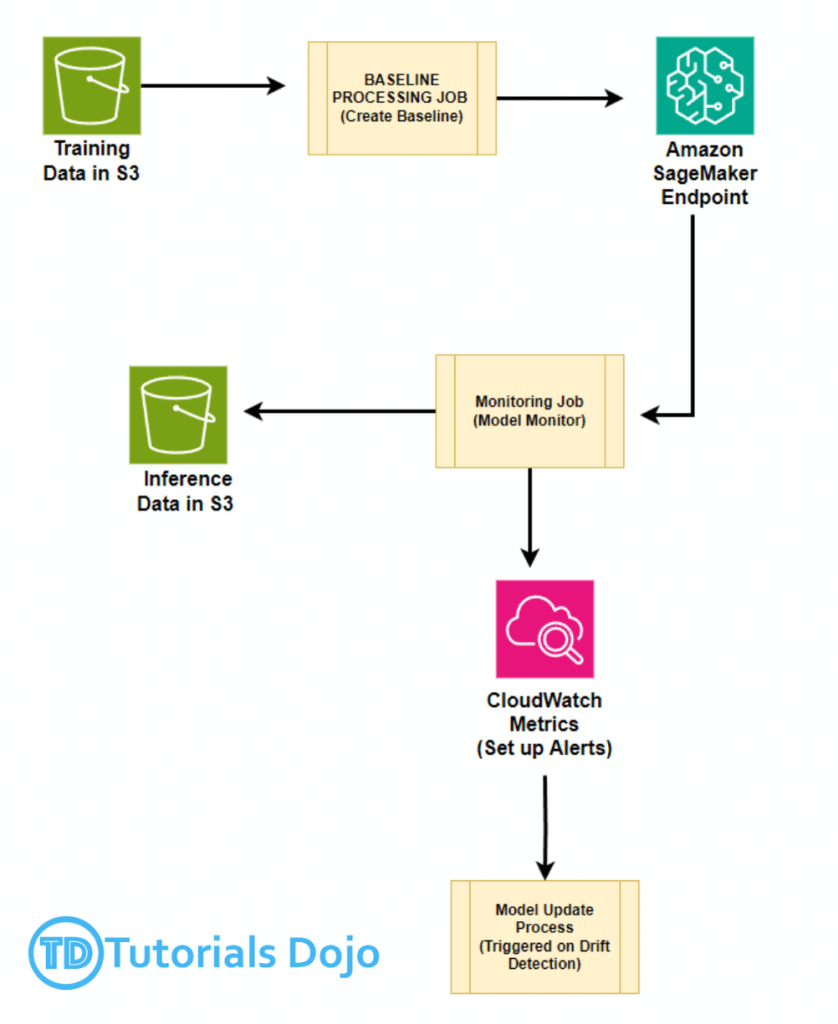
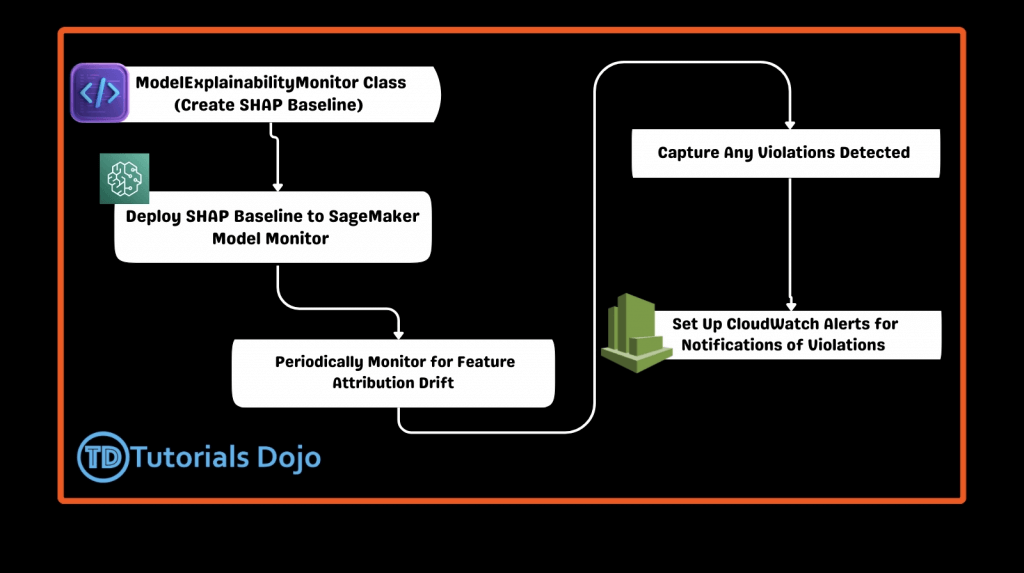
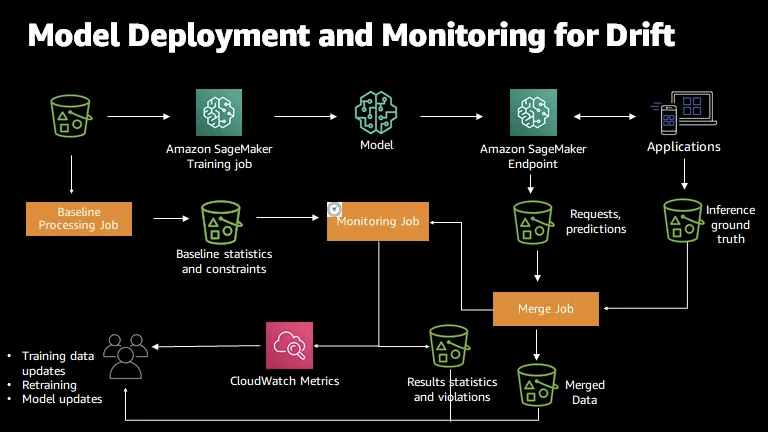
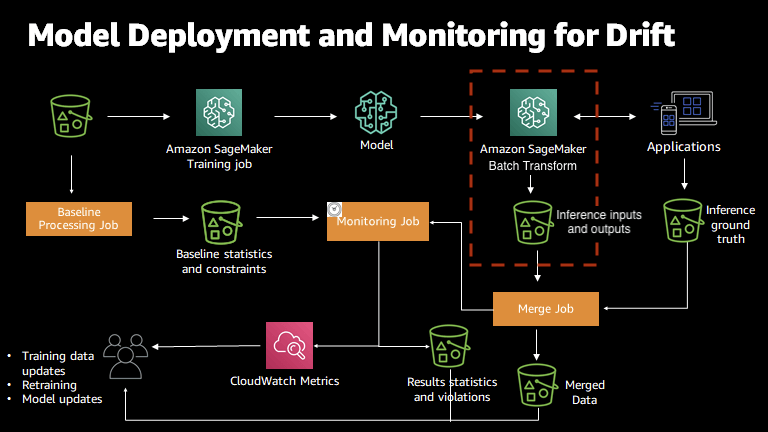
Drift Types | Meaning | Activity | Class |
---|---|---|---|
Data quality drift | production data distribution differs from that used for training – the statistical properties of input data change | missing values or errors in the data | ModelDataQualityMonitor; DefaultModelMonitor |
Model quality drift | predictions that a model makes differ from actual Ground Truth labels that the model attempts to predict | monitor the performance of a model by comparing the predictions that the model makes with the actual ground truth labels that the model attempts to predict | ModelPerformanceMonitor |
Bias drift | introduction of bias due to change in production data distribution or application – starts to favor specific groups over others because of changes in data distribution or model parameters | statistical changes in the data distribution, even if the data has high quality | ModelBiasMonitor |
Feature attribution drift | ranking of individual features changed from training data to live data – the significance of various features in a predictive model changes | detect feature attribution drift by comparing how the ranking of the individual features changed from training data to live data | ModelExplainabilityMonitor |
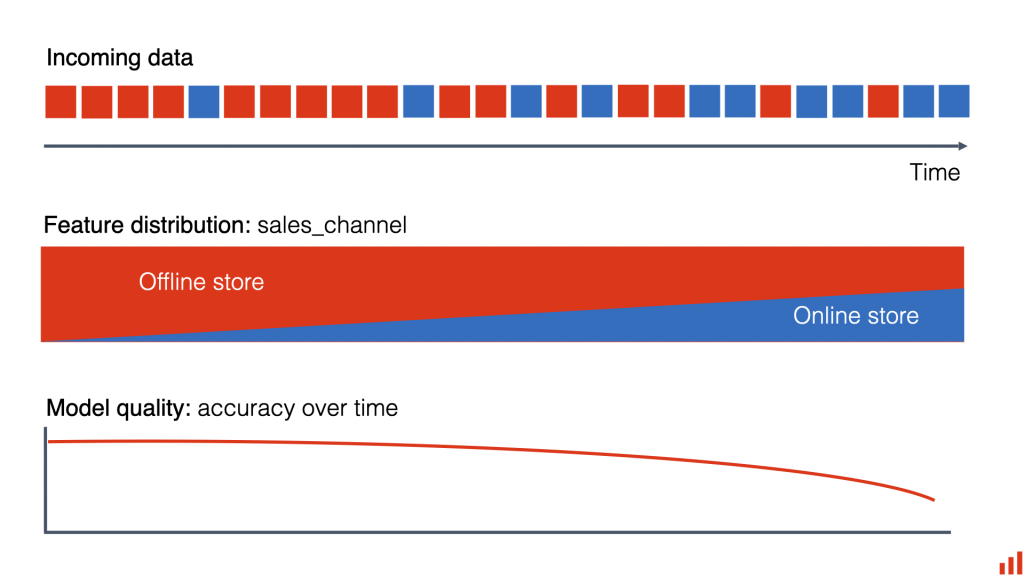
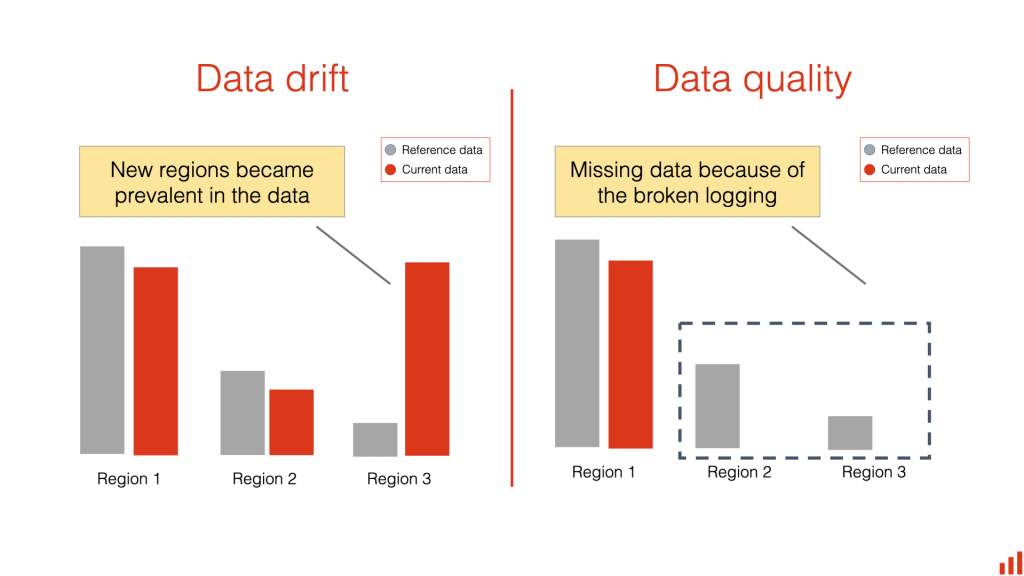
SageMaker Clarify
- Analyze data and models for bias and explainability
- Explainablity: the ability to detail which features contribute the most to a model prediction for a specific input, or feature attribution. These details can help determine if a particular model input has more influence than expected on overall model behaviour.
- detects potential bias, i.e., imbalances across different groups / ages / income brackets
- “Biases in the training data can lead to inaccurate predictions”
- works as “diagnostic” tool, could directly address the root cause of inaccuracies in the training data or model
- help you understand why your ML model made a specific prediction
- and whether bias impacted this prediction during training or inference
- Also provides tools to help build less biased and more understandable models
- and can generate model governance reports for risk and compliance teams and external regulators.
- To setup a SageMaker Clarify processing job, for configuring the processing container, job inputs, outputs, resources, and other parameters
- OPTION ONE: use the SageMaker CreateProcessingJob API
- OPTION TWO: the SageMaker Python SDK API, SageMaker ClarifyProcessor
- With Model Monitor, you can monitor for bias and be alerted to new potential bias via CloudWatch
- SageMaker Clarify also helps explain model behavior
- Understand which features contribute the most to your predictions
- analyzes the model’s predictions on inference data and explains
- Pre-training Bias Metrics
- to identify and address potential biases within a dataset before the model is trained
- Class Imbalance (CI)
- One facet (demographic group) has fewer training values than another
- Difference in Proportions of Labels (DPL)
- Imbalance of positive outcomes between facet values
- Kullback-Leibler Divergence (KL), Jensen-Shannon Divergence(JS)
- How much outcome distributions of facets diverge
- Lp-norm (LP)
- P-norm difference between distributions of outcomes from facets
- Total Variation Distance (TVD)
- L1-norm difference between distributions of outcomes from facets
- Kolmogorov-Smirnov (KS)
- Maximum divergence between outcomes in distributions from facets
- Conditional Demographic Disparity (CDD)
- Disparity of outcomes between facets as a whole, and by subgroups
Bias metric | Description | Example question | Futher Example |
---|---|---|---|
Class Imbalance (CI) | Measures the imbalance in the number of members between different facet values. | Could there be age-based biases due to not having enough data for the demographic outside a middle-aged facet? | For instance, if one gender is significantly underrepresented, CI will reveal this imbalance, which could lead to biased predictions that favor the more represented class. Addressing such imbalances is important to ensure that the model learns fairly from all data groups. |
Difference in Proportions of Labels (DPL) | Measures the imbalance of positive outcomes between different facet values. | Could there be age-based biases in ML predictions due to biased labeling of facet values in the data? | For example, if a model predicts higher success rates for one racial group compared to another, DPL will highlight this discrepancy, indicating a potential bias in outcomes. Analyzing DPL can make adjustments to the model or dataset to reduce such disparities and promote fairness. |
Kullback-Leibler Divergence (KL) | Measures how much the outcome distributions of different facets diverge from each other entropically. primarily measures the divergence between two probability distributions often used to compare the distribution of outcomes across different groups | How different are the distributions for loan application outcomes for different demographic groups? | typically used in broader contexts of distribution comparisons and might not provide a direct assessment of bias related to demographic attributes. |
Jensen-Shannon Divergence (JS) | Measures how much the outcome distributions of different facets diverge from each other entropically. primarily measures the divergence between two probability distributions often used to compare the distribution of outcomes across different groups | How different are the distributions for loan application outcomes for different demographic groups? | typically used in broader contexts of distribution comparisons and might not provide a direct assessment of bias related to demographic attributes. |
Lp-norm (LP) | Measures a p-norm difference between distinct demographic distributions of the outcomes associated with different facets in a dataset. | How different are the distributions for loan application outcomes for different demographics? | It’s a critical metric for identifying how much the predicted outcomes for one group differ from another, thus helping to assess bias in the model’s predictions. |
Total Variation Distance (TVD) | Measures half of the L1-norm difference between distinct demographic distributions of the outcomes associated with different facets in a dataset. TVD quantifies the divergence in outcome distributions between different demographic groups | How different are the distributions for loan application outcomes for different demographics? | It’s a critical metric for identifying how much the predicted outcomes for one group differ from another, thus helping to assess bias in the model’s predictions. – Total Variation Distance (TVD) is a metric primarily used to measure the overall difference between two probability distributions. |
Kolmogorov-Smirnov (KS) | Measures maximum divergence between outcomes in distributions for different facets in a dataset. | Which college application outcomes manifest the greatest disparities by demographic group? | It’s a critical metric for identifying how much the predicted outcomes for one group differ from another, thus helping to assess bias in the model’s predictions. – Kullback-Leibler Divergence (KL) is a metric typically used to measure the difference between two probability distributions, similar to Total Variation Distance. |
Conditional Demographic Disparity (CDD) | Measures the disparity of outcomes between different facets as a whole, but also by subgroups. | Do some groups have a larger proportion of rejections for college admission outcomes than their proportion of acceptances? | It’s a critical metric for identifying how much the predicted outcomes for one group differ from another, thus helping to assess bias in the model’s predictions. |
Explainability | Meaning | Activity |
---|---|---|
Shapley Value | quantify the contribution of each player (features) to a game (prediction of the model), and hence the means to distribute the total gain generated by a game to its players based on their contributions. | use Shapley values to determine the contribution that each feature made to model predictions. These attributions can be provided for specific predictions and at a global level for the model as a whole |
partial dependence plots (PDPs) | Identify the difference in the predicted outcome as an input feature changes. | show the marginal effect features have on the predicted outcome of a machine learning model. Partial dependence helps explain target response given a set of input features – PDPs can be used to “identify how the predicted probability of churn changes as customer age increases.” This technique can help the team understand the relationship between customer age and the predicted churn probability, which can be useful for identifying potential biases or patterns in the model’s predictions. “check the difference in the prediction output(y) as ONE input feature(x) value changes” |
Difference in Proportions of Labels (DPL) | compares the proportion of observed outcomes with positive labels for facet d with the proportion of observed outcomes with positive labels of facet a in a training dataset. | Measure the imbalance of positive outcomes between different facet values. – DPL can be used to “measure the imbalance in the predicted churn rates between different customer demographic groups (e.g., age groups).” This technique can help the team identify potential biases or disparities in the model’s predictions across different demographic groups, which is a crucial consideration for ensuring fairness and avoiding discrimination. “check the difference in the prediction output(y) in different categories/labels” |
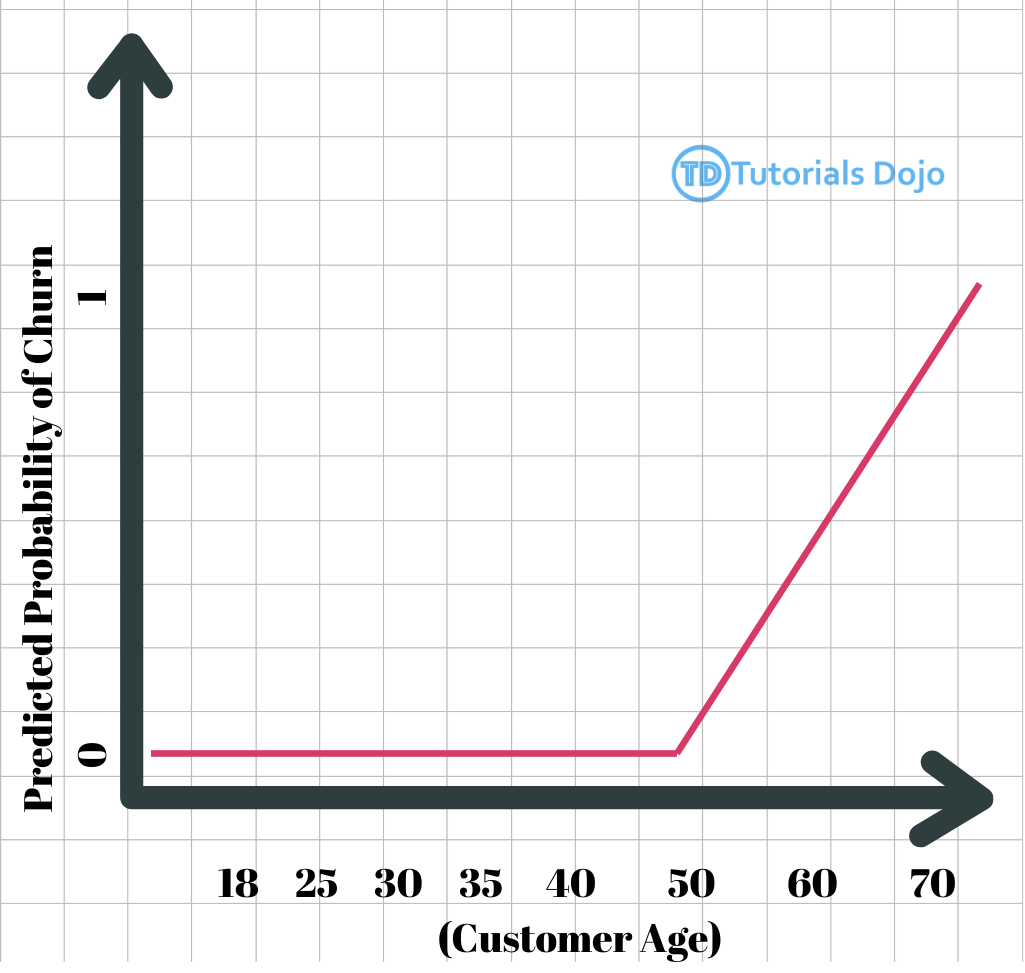
— Model Improvement & Debug —
SageMaker with TensorBoard
- TensorBoard is useful for visualizing training metrics such as accuracy, loss, and gradients, it is primarily a tool for manual inspection rather than real-time, automated monitoring.
- visualize and analyze intermediate tensors during model training. SageMaker with TensorBoard provides full visibility into the model training process, including debugging and model optimization. This solution gives you the ability to debug issues, including lower than expected precision for a specific class.
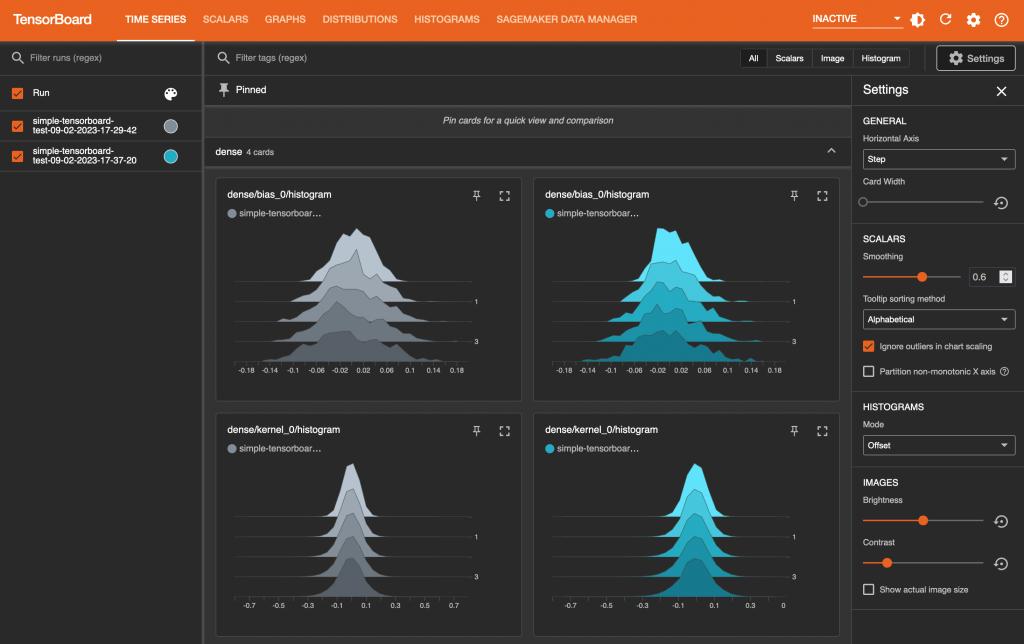
SageMaker Debugger
- used to monitor and debug machine learning training jobs in real-time
- automatically generate alerts when anomalies or specific conditions, such as class imbalances or overfitting, occur during the training
- detecting data imbalances, vanishing gradients, or divergence
- Saves internal model state at periodical intervals
- Gradients / tensors over time as a model is trained
- Define rules for detecting unwanted conditions while training
- A debug job is run for each rule you configure
- Logs & fires a CloudWatch event when the rule is hit
- Auto-generated training reports
- Built-in rules:
- Monitor system bottlenecks
- Profile model framework operations
- Debug model parameters
- Supported Frameworks & Algorithms:
- Tensorflow
- PyTorch
- MXNet
- XGBoost
- SageMaker generic estimator (for use with custom training containers)
- Debugger API’s available in GitHub
- Construct hooks & rules for CreateTrainingJob and DescribeTrainingJob API’s
- SMDebug client library lets you register hooks for accessing training data
- Debugger ProfilerRule
- ProfilerReport
- Hardware system metrics (CPUBottlenck, GPUMemoryIncrease, etc)
- Framework Metrics (MaxInitializationTime, OverallFrameworkMetrics, StepOutlier)
- Built-in actions to receive notifications or stop training
- StopTraining(), Email(), or SMS()
- In response to Debugger Rules
- Sends notifications via SNS
- Profiling system resource usage and training